Conversation Analytics
Conversation Analytics
Conversation Analytics
Conversation Analytics
Conversation Intelligence: What It Is and Why You Need It
Conversation Intelligence: What It Is and Why You Need It
Conversation Intelligence: What It Is and Why You Need It
Conversation Intelligence: What It Is and Why You Need It
|
|
|
|
Oct 16, 2023
Oct 16, 2023
Oct 16, 2023
Oct 16, 2023
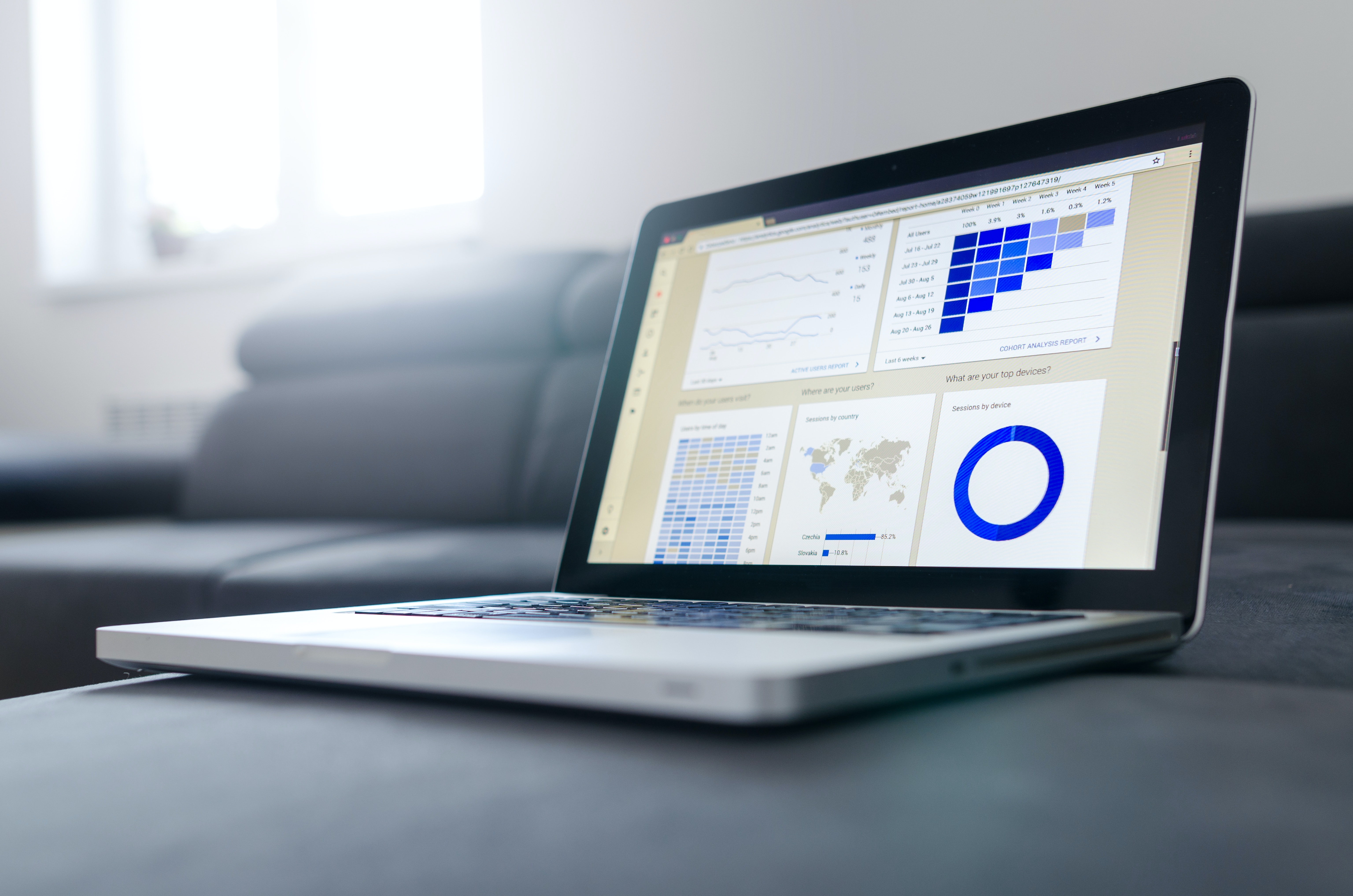
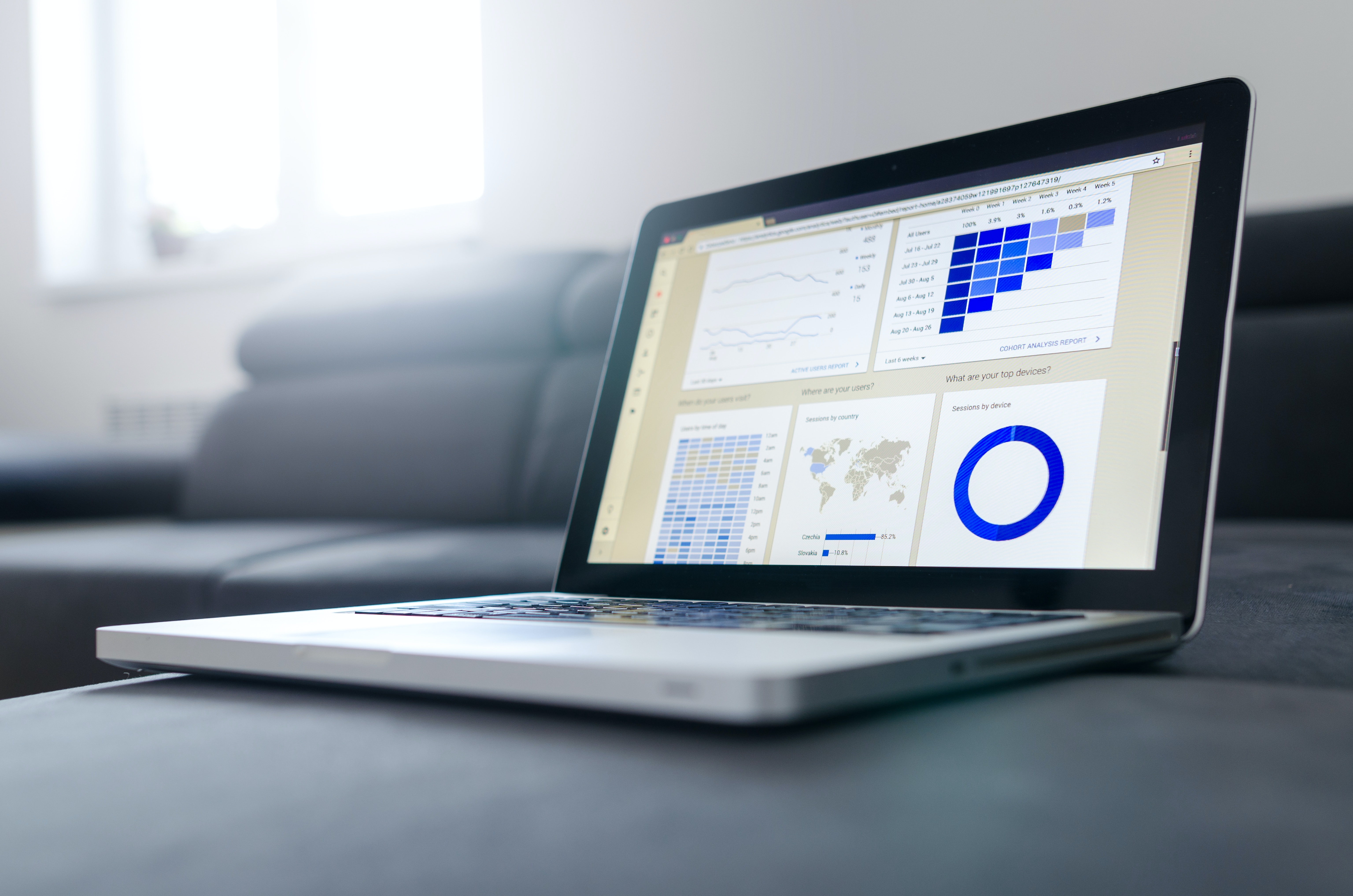
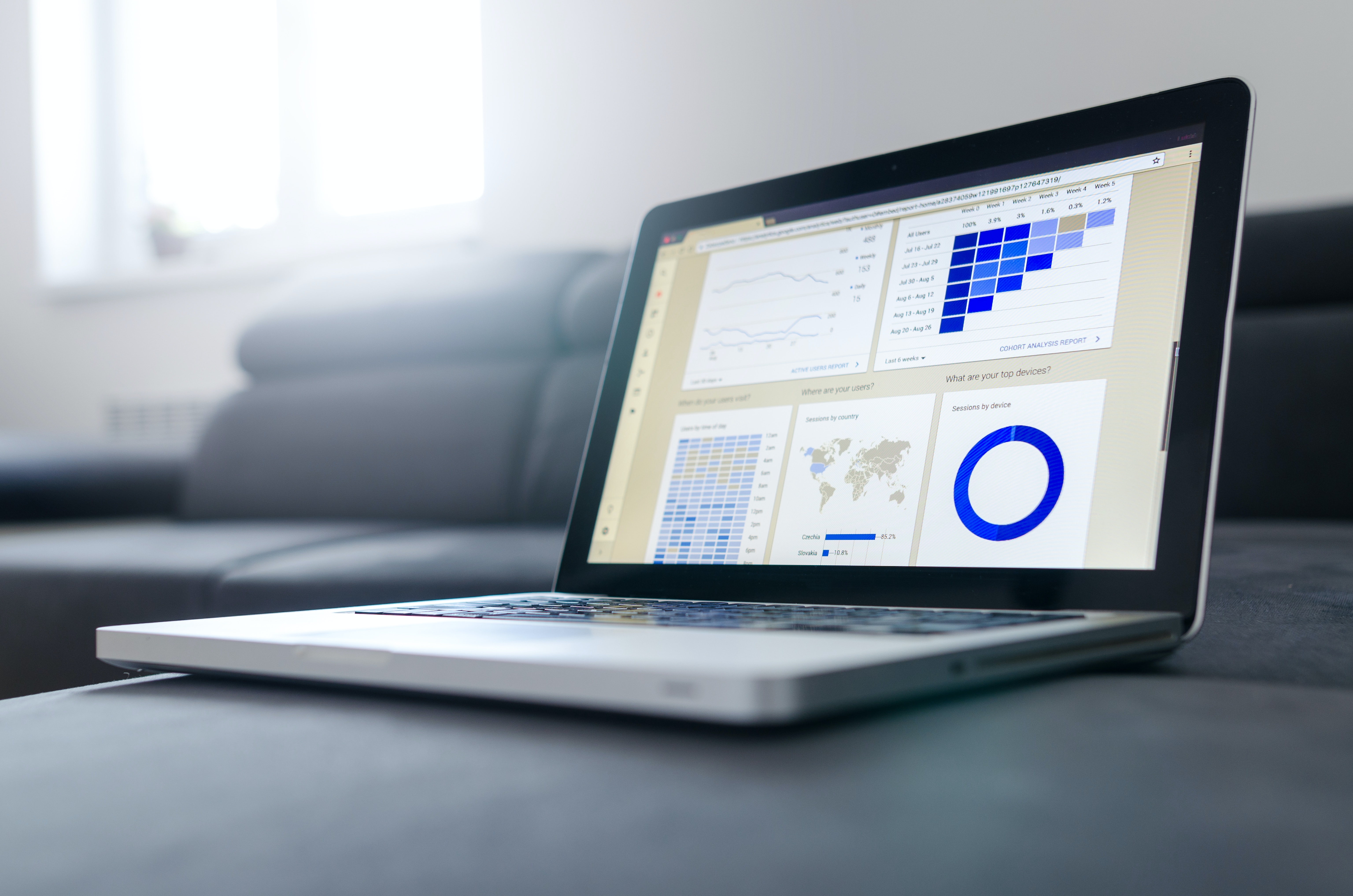
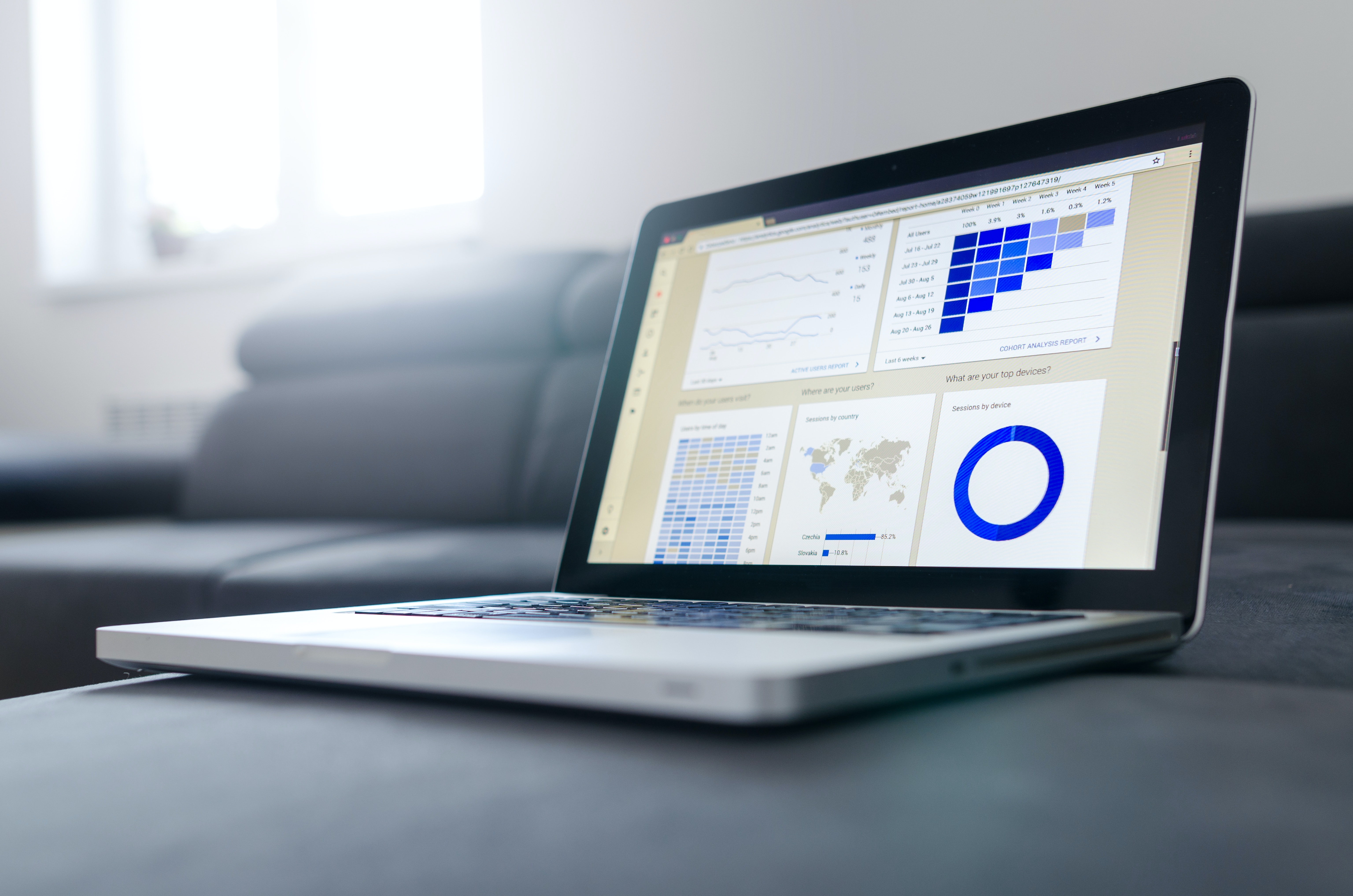
Every customer conversation — whether it's a sales call, service ticket, or support chat — is a rich source of insights into consumer sentiment and product feedback. According to Gartner, 84% of customer service leaders believe customer data and analytics are important to achieve organizational objectives.
If your contact center handles only a few calls, your team might review each interaction and improve the metrics for your contact center. But as call volumes increase, manually sourcing insights can be time-consuming and labor-intensive. That's where conversation intelligence (CI) comes into play.
Conversation intelligence has the capacity to turn everyday dialogues into invaluable insights.
Every customer conversation — whether it's a sales call, service ticket, or support chat — is a rich source of insights into consumer sentiment and product feedback. According to Gartner, 84% of customer service leaders believe customer data and analytics are important to achieve organizational objectives.
If your contact center handles only a few calls, your team might review each interaction and improve the metrics for your contact center. But as call volumes increase, manually sourcing insights can be time-consuming and labor-intensive. That's where conversation intelligence (CI) comes into play.
Conversation intelligence has the capacity to turn everyday dialogues into invaluable insights.
Every customer conversation — whether it's a sales call, service ticket, or support chat — is a rich source of insights into consumer sentiment and product feedback. According to Gartner, 84% of customer service leaders believe customer data and analytics are important to achieve organizational objectives.
If your contact center handles only a few calls, your team might review each interaction and improve the metrics for your contact center. But as call volumes increase, manually sourcing insights can be time-consuming and labor-intensive. That's where conversation intelligence (CI) comes into play.
Conversation intelligence has the capacity to turn everyday dialogues into invaluable insights.
Every customer conversation — whether it's a sales call, service ticket, or support chat — is a rich source of insights into consumer sentiment and product feedback. According to Gartner, 84% of customer service leaders believe customer data and analytics are important to achieve organizational objectives.
If your contact center handles only a few calls, your team might review each interaction and improve the metrics for your contact center. But as call volumes increase, manually sourcing insights can be time-consuming and labor-intensive. That's where conversation intelligence (CI) comes into play.
Conversation intelligence has the capacity to turn everyday dialogues into invaluable insights.
Table of Contents
Table of Contents
Table of Contents
Table of Contents
What Is Conversation Intelligence?
Conversation intelligence gathers and interprets customer interactions across various communication channels. Relying on artificial intelligence and machine learning, conversation intelligence tools perform three broad functions:
First, they capture information from spoken and written conversations, even when they're messy and unorganized.
Then, they match this information with structured metadata about the interaction.
They also apply AI models to analyze customer intent to surface desires, needs, opinions, and expectations of customers.
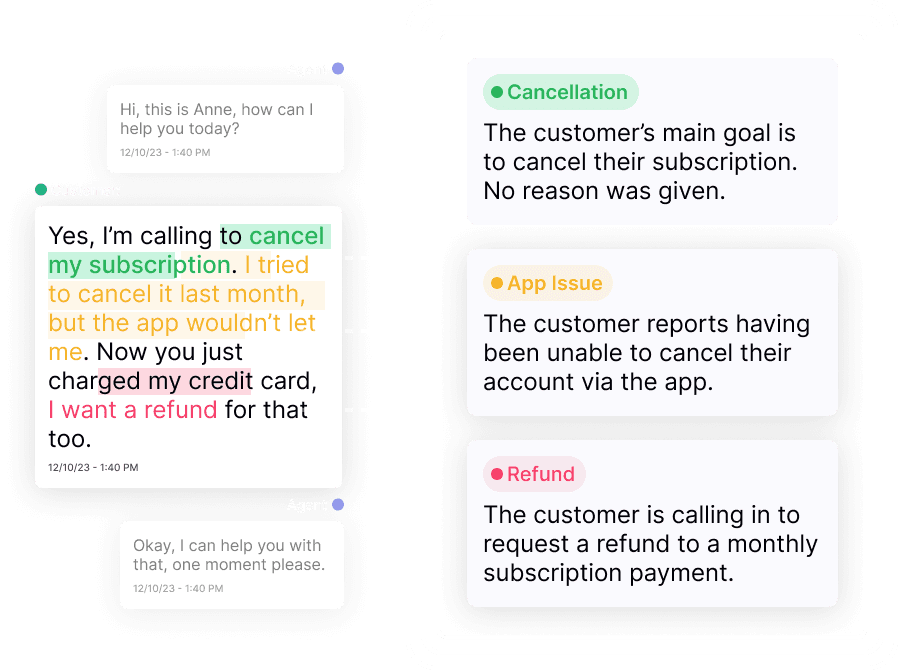
Let's explore this last point around how AI analyzes customer intent, or the reason behind why a customer shares feedback or expresses an unmet need. Not all conversation intelligence tools analyze data the same way. In fact, with new developments in technologies like generative AI (genAI), conversation intelligence tools can now be trained on very large data sets, called Large Language Models (LLMs), which can be used to surface more accurate and trustworthy insights.
Think about this as the difference between a mid-tier athlete and an Olympian—the amount of effort, training, and quality of this training is simply at an entirely different level. That's the difference between classic conversation intelligence insights, and those that are surfaced with genAI.
Further in this blog, we will explore how genAI paired with conversation intelligence is an incredible combination that's shaping a new type of insights.
Conversation intelligence gathers and interprets customer interactions across various communication channels. Relying on artificial intelligence and machine learning, conversation intelligence tools perform three broad functions:
First, they capture information from spoken and written conversations, even when they're messy and unorganized.
Then, they match this information with structured metadata about the interaction.
They also apply AI models to analyze customer intent to surface desires, needs, opinions, and expectations of customers.
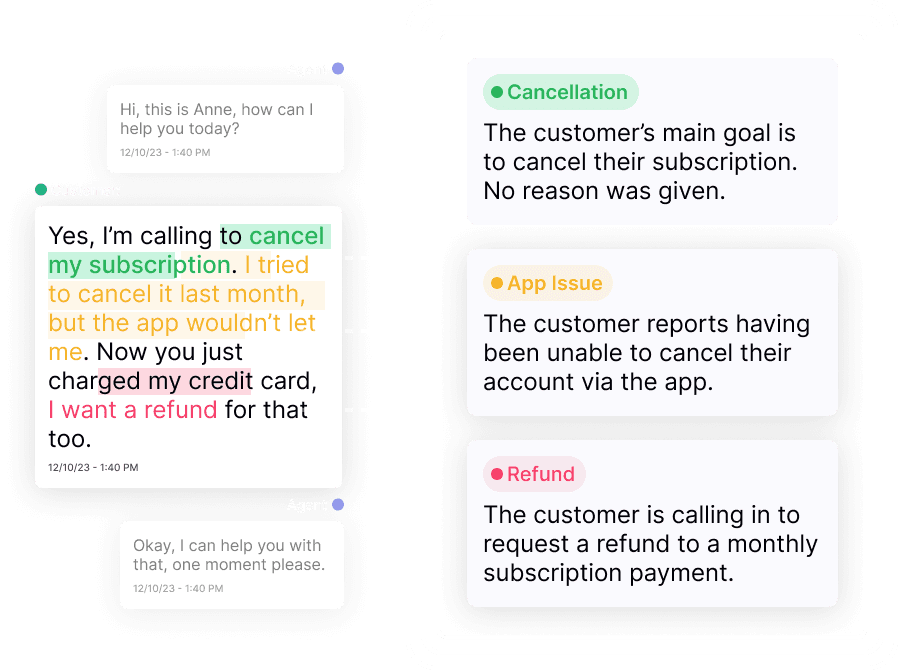
Let's explore this last point around how AI analyzes customer intent, or the reason behind why a customer shares feedback or expresses an unmet need. Not all conversation intelligence tools analyze data the same way. In fact, with new developments in technologies like generative AI (genAI), conversation intelligence tools can now be trained on very large data sets, called Large Language Models (LLMs), which can be used to surface more accurate and trustworthy insights.
Think about this as the difference between a mid-tier athlete and an Olympian—the amount of effort, training, and quality of this training is simply at an entirely different level. That's the difference between classic conversation intelligence insights, and those that are surfaced with genAI.
Further in this blog, we will explore how genAI paired with conversation intelligence is an incredible combination that's shaping a new type of insights.
Conversation intelligence gathers and interprets customer interactions across various communication channels. Relying on artificial intelligence and machine learning, conversation intelligence tools perform three broad functions:
First, they capture information from spoken and written conversations, even when they're messy and unorganized.
Then, they match this information with structured metadata about the interaction.
They also apply AI models to analyze customer intent to surface desires, needs, opinions, and expectations of customers.
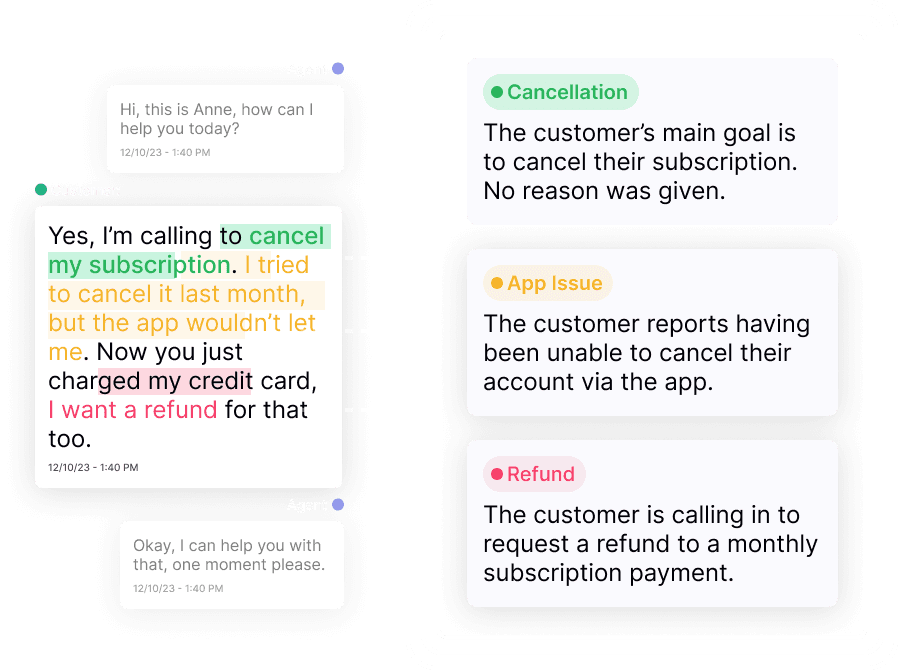
Let's explore this last point around how AI analyzes customer intent, or the reason behind why a customer shares feedback or expresses an unmet need. Not all conversation intelligence tools analyze data the same way. In fact, with new developments in technologies like generative AI (genAI), conversation intelligence tools can now be trained on very large data sets, called Large Language Models (LLMs), which can be used to surface more accurate and trustworthy insights.
Think about this as the difference between a mid-tier athlete and an Olympian—the amount of effort, training, and quality of this training is simply at an entirely different level. That's the difference between classic conversation intelligence insights, and those that are surfaced with genAI.
Further in this blog, we will explore how genAI paired with conversation intelligence is an incredible combination that's shaping a new type of insights.
Conversation intelligence gathers and interprets customer interactions across various communication channels. Relying on artificial intelligence and machine learning, conversation intelligence tools perform three broad functions:
First, they capture information from spoken and written conversations, even when they're messy and unorganized.
Then, they match this information with structured metadata about the interaction.
They also apply AI models to analyze customer intent to surface desires, needs, opinions, and expectations of customers.
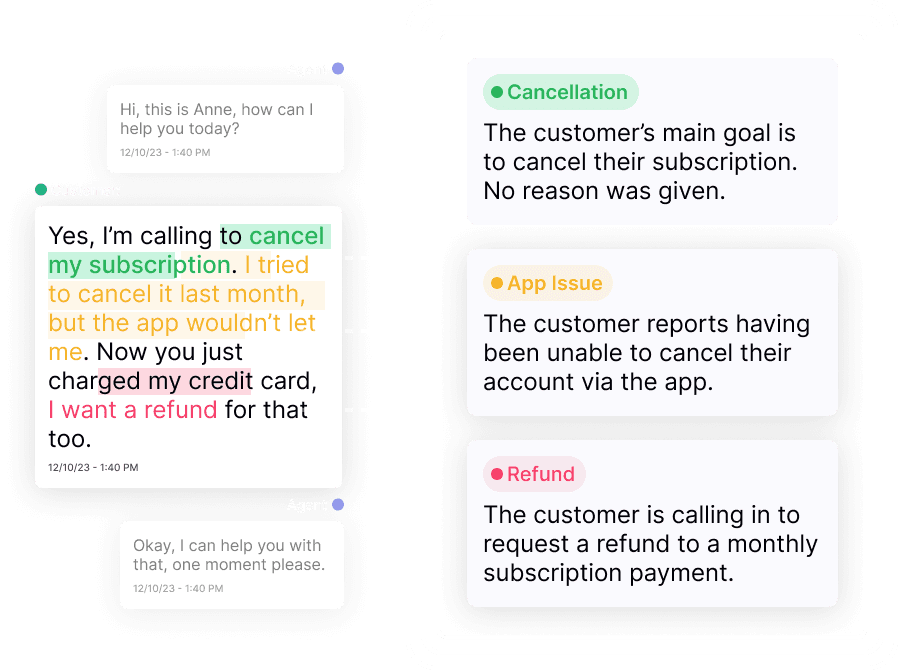
Let's explore this last point around how AI analyzes customer intent, or the reason behind why a customer shares feedback or expresses an unmet need. Not all conversation intelligence tools analyze data the same way. In fact, with new developments in technologies like generative AI (genAI), conversation intelligence tools can now be trained on very large data sets, called Large Language Models (LLMs), which can be used to surface more accurate and trustworthy insights.
Think about this as the difference between a mid-tier athlete and an Olympian—the amount of effort, training, and quality of this training is simply at an entirely different level. That's the difference between classic conversation intelligence insights, and those that are surfaced with genAI.
Further in this blog, we will explore how genAI paired with conversation intelligence is an incredible combination that's shaping a new type of insights.
How Conversation Intelligence Works
The first step involves taking call recordings and running this data through speech-to-text AI models, followed by analyzing text data from emails, chats, surveys, social feeds, and more to make sense of the context of the conversations. This combined use of machine learning and natural language processing technology is conversation intelligence.
Let's explore the six core components of conversation intelligence, and what you need to know in a nutshell about the capabilities it offers.
Speech-to-text conversion: Conversation intelligence begins by converting spoken words into written text. Advanced speech recognition technology ensures accuracy, even in noisy environments or with multiple speakers.
Sentiment analysis: This component gauges the emotional tone of a conversation. It can determine whether the participants are happy, frustrated, satisfied, or dissatisfied. Understanding sentiment is crucial for improving customer experiences.
Keyword and phrase recognition: Conversation AI tools identify specific keywords and phrases discussed during conversations to support teams looking for common customer issues.
Call summarization: Instead of sifting through hours of recorded conversations, conversation artificial intelligence helps to provide concise summaries and highlight key takeaways.
Performance metrics: Contact centers can track and measure key performance indicators (KPIs) related to conversations, such as call duration, response time, or customer satisfaction scores.
Integration with CRM systems: Many CI tools integrate with customer relationship management (CRM) systems, ensuring that insights from conversations are directly linked to customer profiles and sales pipelines. They can also write back into the CRM to further enrich the data.
The first step involves taking call recordings and running this data through speech-to-text AI models, followed by analyzing text data from emails, chats, surveys, social feeds, and more to make sense of the context of the conversations. This combined use of machine learning and natural language processing technology is conversation intelligence.
Let's explore the six core components of conversation intelligence, and what you need to know in a nutshell about the capabilities it offers.
Speech-to-text conversion: Conversation intelligence begins by converting spoken words into written text. Advanced speech recognition technology ensures accuracy, even in noisy environments or with multiple speakers.
Sentiment analysis: This component gauges the emotional tone of a conversation. It can determine whether the participants are happy, frustrated, satisfied, or dissatisfied. Understanding sentiment is crucial for improving customer experiences.
Keyword and phrase recognition: Conversation AI tools identify specific keywords and phrases discussed during conversations to support teams looking for common customer issues.
Call summarization: Instead of sifting through hours of recorded conversations, conversation artificial intelligence helps to provide concise summaries and highlight key takeaways.
Performance metrics: Contact centers can track and measure key performance indicators (KPIs) related to conversations, such as call duration, response time, or customer satisfaction scores.
Integration with CRM systems: Many CI tools integrate with customer relationship management (CRM) systems, ensuring that insights from conversations are directly linked to customer profiles and sales pipelines. They can also write back into the CRM to further enrich the data.
The first step involves taking call recordings and running this data through speech-to-text AI models, followed by analyzing text data from emails, chats, surveys, social feeds, and more to make sense of the context of the conversations. This combined use of machine learning and natural language processing technology is conversation intelligence.
Let's explore the six core components of conversation intelligence, and what you need to know in a nutshell about the capabilities it offers.
Speech-to-text conversion: Conversation intelligence begins by converting spoken words into written text. Advanced speech recognition technology ensures accuracy, even in noisy environments or with multiple speakers.
Sentiment analysis: This component gauges the emotional tone of a conversation. It can determine whether the participants are happy, frustrated, satisfied, or dissatisfied. Understanding sentiment is crucial for improving customer experiences.
Keyword and phrase recognition: Conversation AI tools identify specific keywords and phrases discussed during conversations to support teams looking for common customer issues.
Call summarization: Instead of sifting through hours of recorded conversations, conversation artificial intelligence helps to provide concise summaries and highlight key takeaways.
Performance metrics: Contact centers can track and measure key performance indicators (KPIs) related to conversations, such as call duration, response time, or customer satisfaction scores.
Integration with CRM systems: Many CI tools integrate with customer relationship management (CRM) systems, ensuring that insights from conversations are directly linked to customer profiles and sales pipelines. They can also write back into the CRM to further enrich the data.
The first step involves taking call recordings and running this data through speech-to-text AI models, followed by analyzing text data from emails, chats, surveys, social feeds, and more to make sense of the context of the conversations. This combined use of machine learning and natural language processing technology is conversation intelligence.
Let's explore the six core components of conversation intelligence, and what you need to know in a nutshell about the capabilities it offers.
Speech-to-text conversion: Conversation intelligence begins by converting spoken words into written text. Advanced speech recognition technology ensures accuracy, even in noisy environments or with multiple speakers.
Sentiment analysis: This component gauges the emotional tone of a conversation. It can determine whether the participants are happy, frustrated, satisfied, or dissatisfied. Understanding sentiment is crucial for improving customer experiences.
Keyword and phrase recognition: Conversation AI tools identify specific keywords and phrases discussed during conversations to support teams looking for common customer issues.
Call summarization: Instead of sifting through hours of recorded conversations, conversation artificial intelligence helps to provide concise summaries and highlight key takeaways.
Performance metrics: Contact centers can track and measure key performance indicators (KPIs) related to conversations, such as call duration, response time, or customer satisfaction scores.
Integration with CRM systems: Many CI tools integrate with customer relationship management (CRM) systems, ensuring that insights from conversations are directly linked to customer profiles and sales pipelines. They can also write back into the CRM to further enrich the data.
Infusing Generative AI into Conversation Intelligence
Now, let's explore what happens when we add generative AI. It's important to understand that even though generative capabilities have been focused on things like generating images, improving live agent assistance, and even fully automating chat responses, the gen AI use case for insights is powerful.
In fact, according to a recent Forrester report, using genAI for the analysis of customer conversation data is "one of the most straightforward applications that offers clear, attributable ROI."
By bringing the power of generative capabilities into data analysis, businesses can sift through millions of customer data points to find risks and opportunities across product, supply chain, marketing, and more.
GenAI-powered CI, or Generative Conversation Intelligence (GenCI) uses multiple large language models, or LLMs, to analyze millions of customer data points at once and extract robust, AI-generated insights on critical business risks and opportunities. Generative CI surfaces topics, themes and keywords by itself at a level of detail that is unmatched by most other technologies on the market.
After raw data has been analyzed through speech-to-text models, the data is routed through a pipeline of numerous LLMs, each designed to tackle a specific action or business question. Some common analysis points include:
Customer intent, or the reason behind why a customer has expressed a frustration, concern, or desire
Customer sentiment, which uncovers instances of negative and positive emotions expressed by the customer
Agent sentiment, which uncovers instances of negative and positive emotions expressed by the agent
Specific details about supply chain, operations, fulfillment, product quality, and more
Once the data has been passed through this second layer of analysis, generative insights surface key trends and themes that have been detected from the data. Generative insights refer to the learnings that have been autonomously detected across millions of data points without any manual tagging or categorization.
These generative insights are summarized by category— providing users with helpful overviews of intent, sentiment, and resolution scores—and organized into high-level themes and trends. With these topic summarizations, business leaders can quickly uncover the reasons behind call surges, cancellations, returns, and more.
The result? Root causes of pressing business issues can be identified faster, even without pre-set keywords or tags.
The significant difference between conversation intelligence and genCI is the elimination of this tedious step of configuration. Most conversation intelligence platforms today still require the manual effort of inputting keywords, tags and user-driven category creation, which places the burden on the user to build an insights infrastructure.
While this is great for already-known issues, it makes it very difficult to proactively find insights that businesses don’t know to look for, leaving hidden insights unseen.
Now, let's explore what happens when we add generative AI. It's important to understand that even though generative capabilities have been focused on things like generating images, improving live agent assistance, and even fully automating chat responses, the gen AI use case for insights is powerful.
In fact, according to a recent Forrester report, using genAI for the analysis of customer conversation data is "one of the most straightforward applications that offers clear, attributable ROI."
By bringing the power of generative capabilities into data analysis, businesses can sift through millions of customer data points to find risks and opportunities across product, supply chain, marketing, and more.
GenAI-powered CI, or Generative Conversation Intelligence (GenCI) uses multiple large language models, or LLMs, to analyze millions of customer data points at once and extract robust, AI-generated insights on critical business risks and opportunities. Generative CI surfaces topics, themes and keywords by itself at a level of detail that is unmatched by most other technologies on the market.
After raw data has been analyzed through speech-to-text models, the data is routed through a pipeline of numerous LLMs, each designed to tackle a specific action or business question. Some common analysis points include:
Customer intent, or the reason behind why a customer has expressed a frustration, concern, or desire
Customer sentiment, which uncovers instances of negative and positive emotions expressed by the customer
Agent sentiment, which uncovers instances of negative and positive emotions expressed by the agent
Specific details about supply chain, operations, fulfillment, product quality, and more
Once the data has been passed through this second layer of analysis, generative insights surface key trends and themes that have been detected from the data. Generative insights refer to the learnings that have been autonomously detected across millions of data points without any manual tagging or categorization.
These generative insights are summarized by category— providing users with helpful overviews of intent, sentiment, and resolution scores—and organized into high-level themes and trends. With these topic summarizations, business leaders can quickly uncover the reasons behind call surges, cancellations, returns, and more.
The result? Root causes of pressing business issues can be identified faster, even without pre-set keywords or tags.
The significant difference between conversation intelligence and genCI is the elimination of this tedious step of configuration. Most conversation intelligence platforms today still require the manual effort of inputting keywords, tags and user-driven category creation, which places the burden on the user to build an insights infrastructure.
While this is great for already-known issues, it makes it very difficult to proactively find insights that businesses don’t know to look for, leaving hidden insights unseen.
Now, let's explore what happens when we add generative AI. It's important to understand that even though generative capabilities have been focused on things like generating images, improving live agent assistance, and even fully automating chat responses, the gen AI use case for insights is powerful.
In fact, according to a recent Forrester report, using genAI for the analysis of customer conversation data is "one of the most straightforward applications that offers clear, attributable ROI."
By bringing the power of generative capabilities into data analysis, businesses can sift through millions of customer data points to find risks and opportunities across product, supply chain, marketing, and more.
GenAI-powered CI, or Generative Conversation Intelligence (GenCI) uses multiple large language models, or LLMs, to analyze millions of customer data points at once and extract robust, AI-generated insights on critical business risks and opportunities. Generative CI surfaces topics, themes and keywords by itself at a level of detail that is unmatched by most other technologies on the market.
After raw data has been analyzed through speech-to-text models, the data is routed through a pipeline of numerous LLMs, each designed to tackle a specific action or business question. Some common analysis points include:
Customer intent, or the reason behind why a customer has expressed a frustration, concern, or desire
Customer sentiment, which uncovers instances of negative and positive emotions expressed by the customer
Agent sentiment, which uncovers instances of negative and positive emotions expressed by the agent
Specific details about supply chain, operations, fulfillment, product quality, and more
Once the data has been passed through this second layer of analysis, generative insights surface key trends and themes that have been detected from the data. Generative insights refer to the learnings that have been autonomously detected across millions of data points without any manual tagging or categorization.
These generative insights are summarized by category— providing users with helpful overviews of intent, sentiment, and resolution scores—and organized into high-level themes and trends. With these topic summarizations, business leaders can quickly uncover the reasons behind call surges, cancellations, returns, and more.
The result? Root causes of pressing business issues can be identified faster, even without pre-set keywords or tags.
The significant difference between conversation intelligence and genCI is the elimination of this tedious step of configuration. Most conversation intelligence platforms today still require the manual effort of inputting keywords, tags and user-driven category creation, which places the burden on the user to build an insights infrastructure.
While this is great for already-known issues, it makes it very difficult to proactively find insights that businesses don’t know to look for, leaving hidden insights unseen.
Now, let's explore what happens when we add generative AI. It's important to understand that even though generative capabilities have been focused on things like generating images, improving live agent assistance, and even fully automating chat responses, the gen AI use case for insights is powerful.
In fact, according to a recent Forrester report, using genAI for the analysis of customer conversation data is "one of the most straightforward applications that offers clear, attributable ROI."
By bringing the power of generative capabilities into data analysis, businesses can sift through millions of customer data points to find risks and opportunities across product, supply chain, marketing, and more.
GenAI-powered CI, or Generative Conversation Intelligence (GenCI) uses multiple large language models, or LLMs, to analyze millions of customer data points at once and extract robust, AI-generated insights on critical business risks and opportunities. Generative CI surfaces topics, themes and keywords by itself at a level of detail that is unmatched by most other technologies on the market.
After raw data has been analyzed through speech-to-text models, the data is routed through a pipeline of numerous LLMs, each designed to tackle a specific action or business question. Some common analysis points include:
Customer intent, or the reason behind why a customer has expressed a frustration, concern, or desire
Customer sentiment, which uncovers instances of negative and positive emotions expressed by the customer
Agent sentiment, which uncovers instances of negative and positive emotions expressed by the agent
Specific details about supply chain, operations, fulfillment, product quality, and more
Once the data has been passed through this second layer of analysis, generative insights surface key trends and themes that have been detected from the data. Generative insights refer to the learnings that have been autonomously detected across millions of data points without any manual tagging or categorization.
These generative insights are summarized by category— providing users with helpful overviews of intent, sentiment, and resolution scores—and organized into high-level themes and trends. With these topic summarizations, business leaders can quickly uncover the reasons behind call surges, cancellations, returns, and more.
The result? Root causes of pressing business issues can be identified faster, even without pre-set keywords or tags.
The significant difference between conversation intelligence and genCI is the elimination of this tedious step of configuration. Most conversation intelligence platforms today still require the manual effort of inputting keywords, tags and user-driven category creation, which places the burden on the user to build an insights infrastructure.
While this is great for already-known issues, it makes it very difficult to proactively find insights that businesses don’t know to look for, leaving hidden insights unseen.
Benefits & How It's Used Today
Conversation Intelligence, and GenCI in particular, can be used to impact every layer of the business, not just the customer service org. From product to marketing to operations, business leaders can take action on key trends to prevent churn and drive growth. Here’s how.
Uncovering hidden customer insights
With analysis across every channel, businesses can get deep, detailed answers to unlock a new level of customer understanding. With generative insights, getting to the bottom of emerging issues is faster and enables customer service teams to more easily know what to do. If a customer expresses discontent around product quality, getting detailed information on the complaint, the specific product, and whether it’s a trend across several feedback channels can be surfaced in seconds.
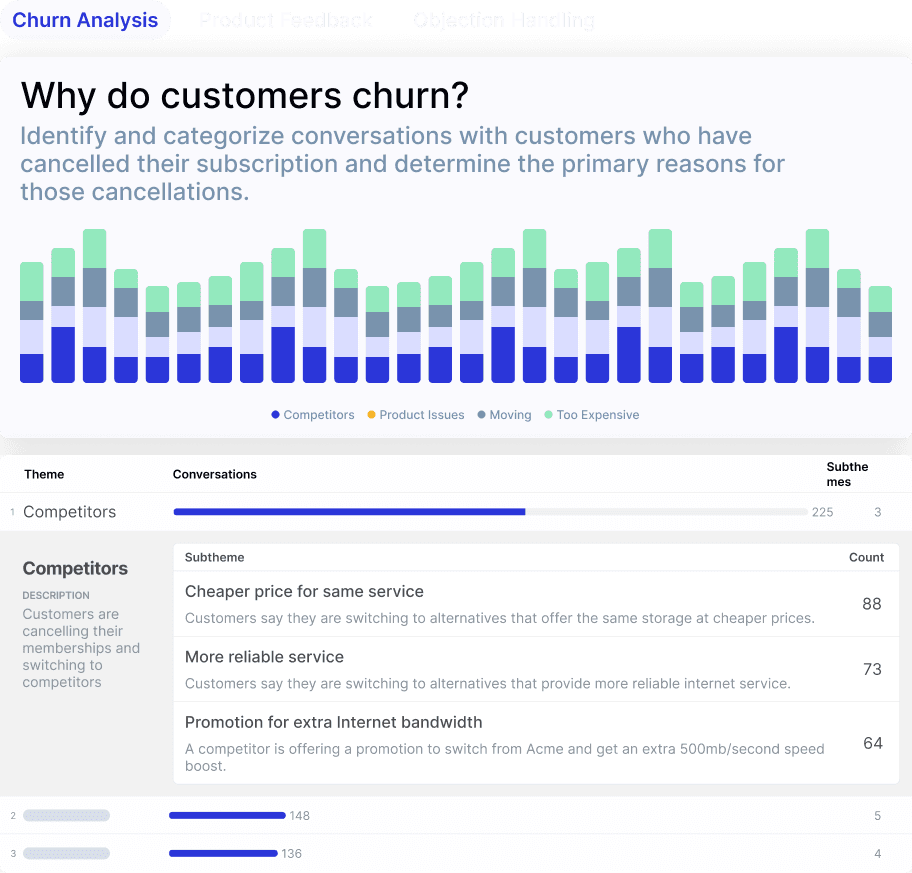
For example, Wine Enthusiast faced a surge in customer inquiries as a result of high demand for their at-home wine delivery services, which was straining their lean customer service team. They needed a better way to analyze customer conversations and act on them in the moment.
Using Echo AI, Wine Enthusiast discovered a trend in customer mentions that led them to discover a manufacturing defect that was causing rusting on electrical components. This insight helped Wine Enthusiast swiftly resolve the manufacturing issue to prevent any future customer churn, ensuring their quality standards were upheld.
Automating Manual Call Reviews
Harnessing AI and automation isn't just about cutting costs—it's about maximizing output.
By automating the most repetitive tasks in a customer service organization, employees can focus on the work that makes the biggest difference, rather than reviewing hundreds of calls manually, for instance.
With Echo AI's AutoQA capabilities, analyzing every customer interaction is completed within minutes. This eliminates the need for manual review, saving businesses valuable time previously spent identifying reasons behind call surges or complaints.
Conversational AI also helps to assess and score agents based on predefined criteria, such as:
Adherence to scripts
Professionalism
Resolution efficiency
Compliance with company policies
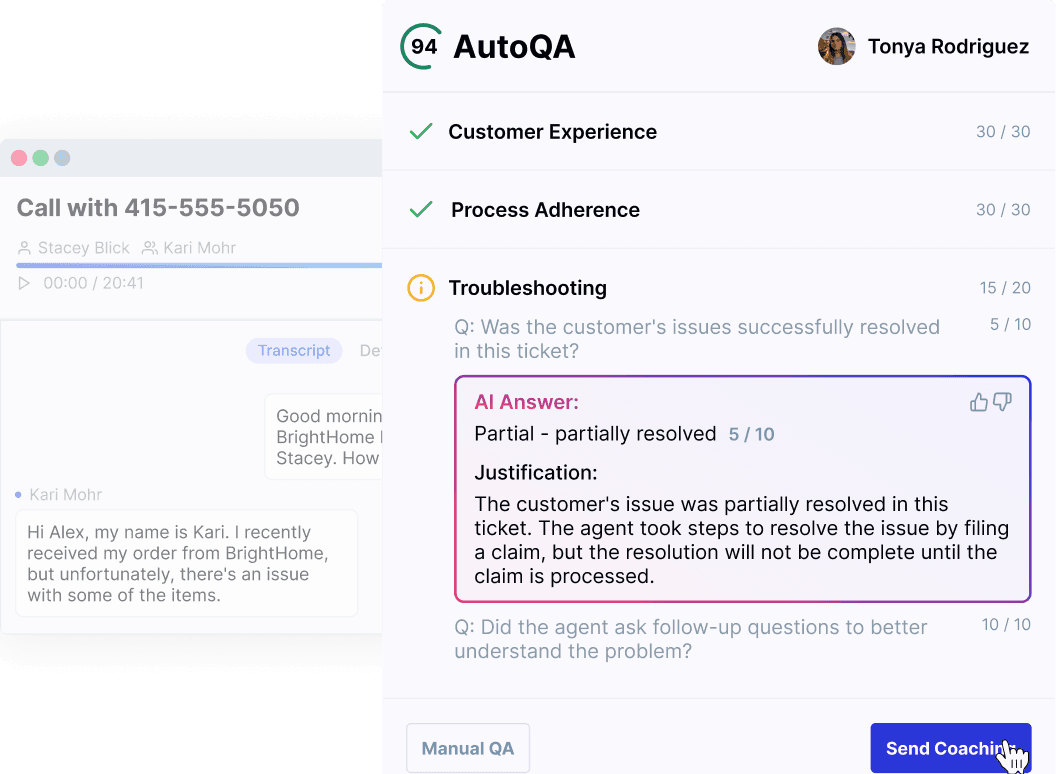
Generative insights are also used to automate quality scoring and act on insights, which also relieves the burden of having to communicate alerts manually across departments.
As an example, NativePath, a health and nutrition brand, was spending 75% of the week manually reviewing calls to glean insights into agent performance. It was a time-consuming process that hindered efficiency and productivity. Now, NativePath has made its review process quicker than ever, reducing time spent by 90% across the board.
Using Insights to Boost Campaigns
The value of genCI goes well beyond the customer service organization. Marketing teams, for example, can gain immense value from using customer data insights. As a function that is deeply tied to understanding the Voice of the Customer, marketing stands to gain immense value from chatbot interactions, calls, support tickets, and more to improve acquisition and lifecycle marketing efforts. (You can get a deeper dive on this here).
Generative insights can be used to improve how audiences are segmented, providing a way to optimize these cohorts at a much more data-driven level. GenCI can identify intent, or the key phrases, recurring topics, and sentiment indicators that reveal customer interests, concerns, and intentions.
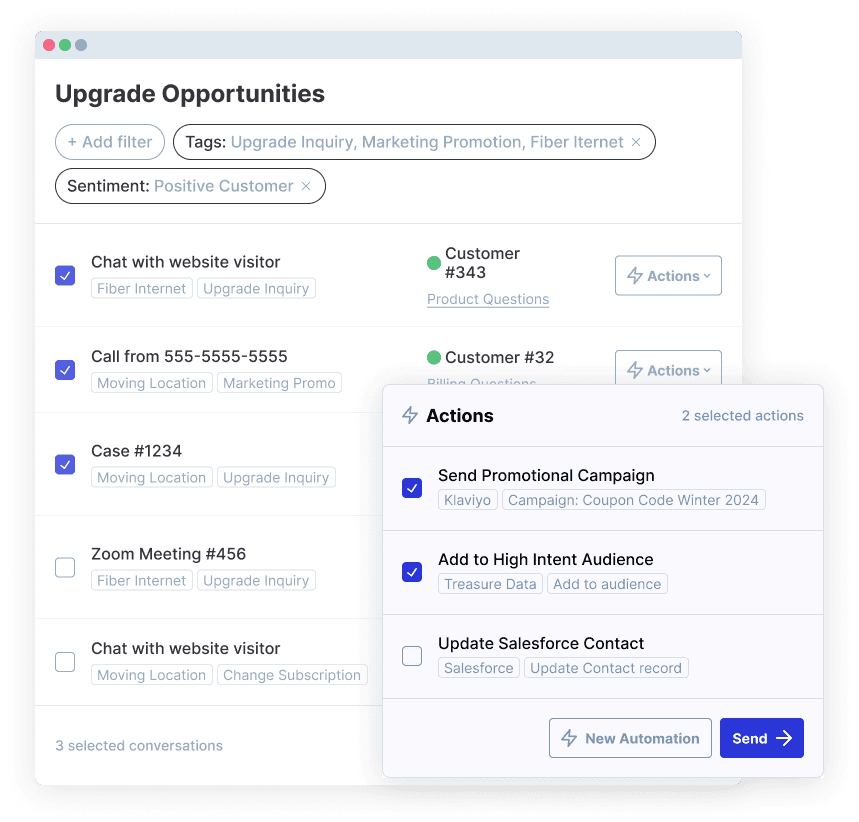
With automatic actions, insights can be integrated into a Customer Data Platform (CDP) to update individual customer profiles. For instance, if a customer frequently asks about a particular product feature, their profile can be tagged to indicate a specific interest in that feature. Using these updated profiles, dynamic audience segments can be created, such as "interested in new products," "requires customer support," or "high purchase intent."
Conversation Intelligence, and GenCI in particular, can be used to impact every layer of the business, not just the customer service org. From product to marketing to operations, business leaders can take action on key trends to prevent churn and drive growth. Here’s how.
Uncovering hidden customer insights
With analysis across every channel, businesses can get deep, detailed answers to unlock a new level of customer understanding. With generative insights, getting to the bottom of emerging issues is faster and enables customer service teams to more easily know what to do. If a customer expresses discontent around product quality, getting detailed information on the complaint, the specific product, and whether it’s a trend across several feedback channels can be surfaced in seconds.
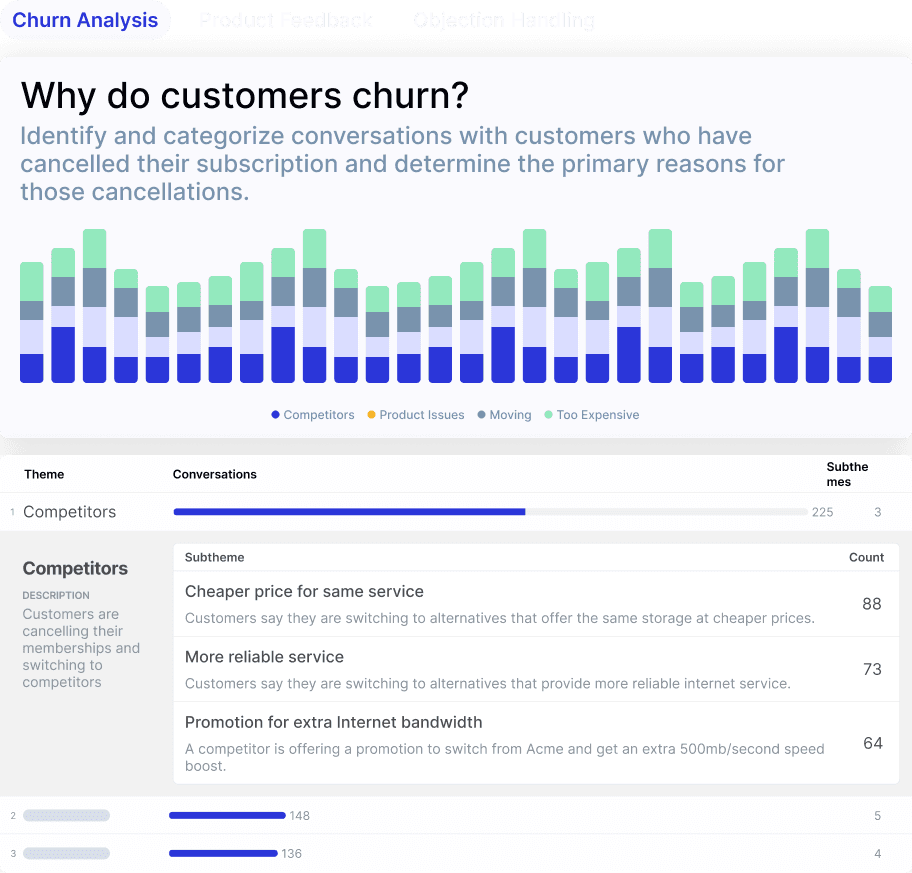
For example, Wine Enthusiast faced a surge in customer inquiries as a result of high demand for their at-home wine delivery services, which was straining their lean customer service team. They needed a better way to analyze customer conversations and act on them in the moment.
Using Echo AI, Wine Enthusiast discovered a trend in customer mentions that led them to discover a manufacturing defect that was causing rusting on electrical components. This insight helped Wine Enthusiast swiftly resolve the manufacturing issue to prevent any future customer churn, ensuring their quality standards were upheld.
Automating Manual Call Reviews
Harnessing AI and automation isn't just about cutting costs—it's about maximizing output.
By automating the most repetitive tasks in a customer service organization, employees can focus on the work that makes the biggest difference, rather than reviewing hundreds of calls manually, for instance.
With Echo AI's AutoQA capabilities, analyzing every customer interaction is completed within minutes. This eliminates the need for manual review, saving businesses valuable time previously spent identifying reasons behind call surges or complaints.
Conversational AI also helps to assess and score agents based on predefined criteria, such as:
Adherence to scripts
Professionalism
Resolution efficiency
Compliance with company policies
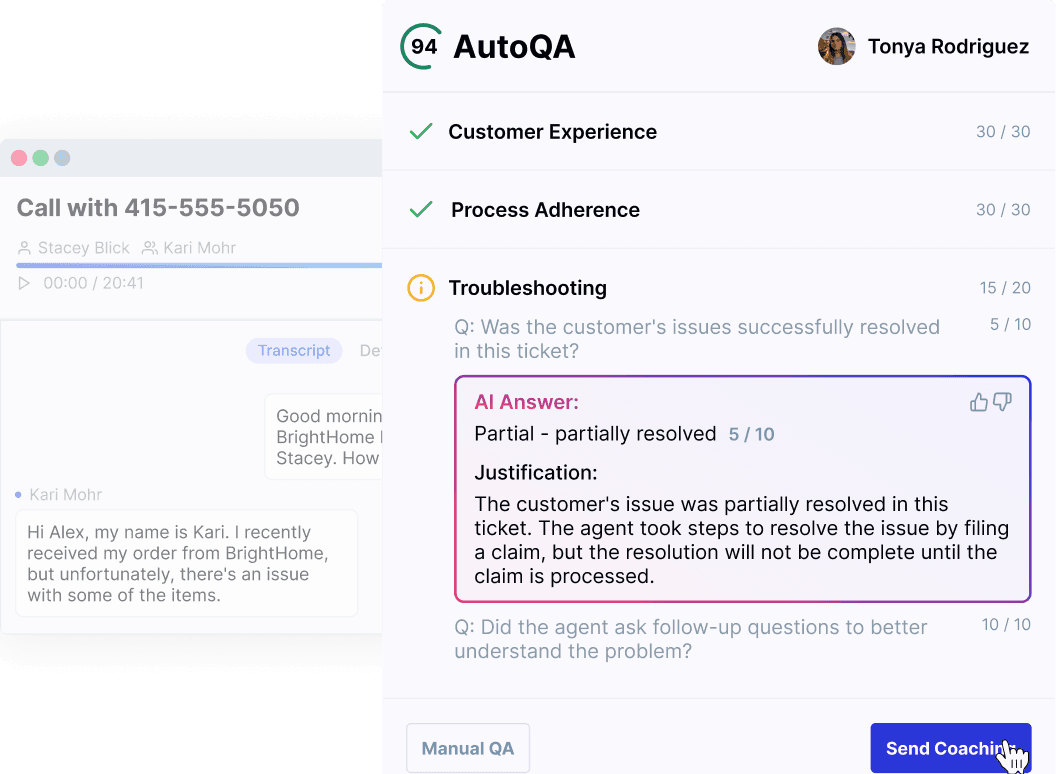
Generative insights are also used to automate quality scoring and act on insights, which also relieves the burden of having to communicate alerts manually across departments.
As an example, NativePath, a health and nutrition brand, was spending 75% of the week manually reviewing calls to glean insights into agent performance. It was a time-consuming process that hindered efficiency and productivity. Now, NativePath has made its review process quicker than ever, reducing time spent by 90% across the board.
Using Insights to Boost Campaigns
The value of genCI goes well beyond the customer service organization. Marketing teams, for example, can gain immense value from using customer data insights. As a function that is deeply tied to understanding the Voice of the Customer, marketing stands to gain immense value from chatbot interactions, calls, support tickets, and more to improve acquisition and lifecycle marketing efforts. (You can get a deeper dive on this here).
Generative insights can be used to improve how audiences are segmented, providing a way to optimize these cohorts at a much more data-driven level. GenCI can identify intent, or the key phrases, recurring topics, and sentiment indicators that reveal customer interests, concerns, and intentions.
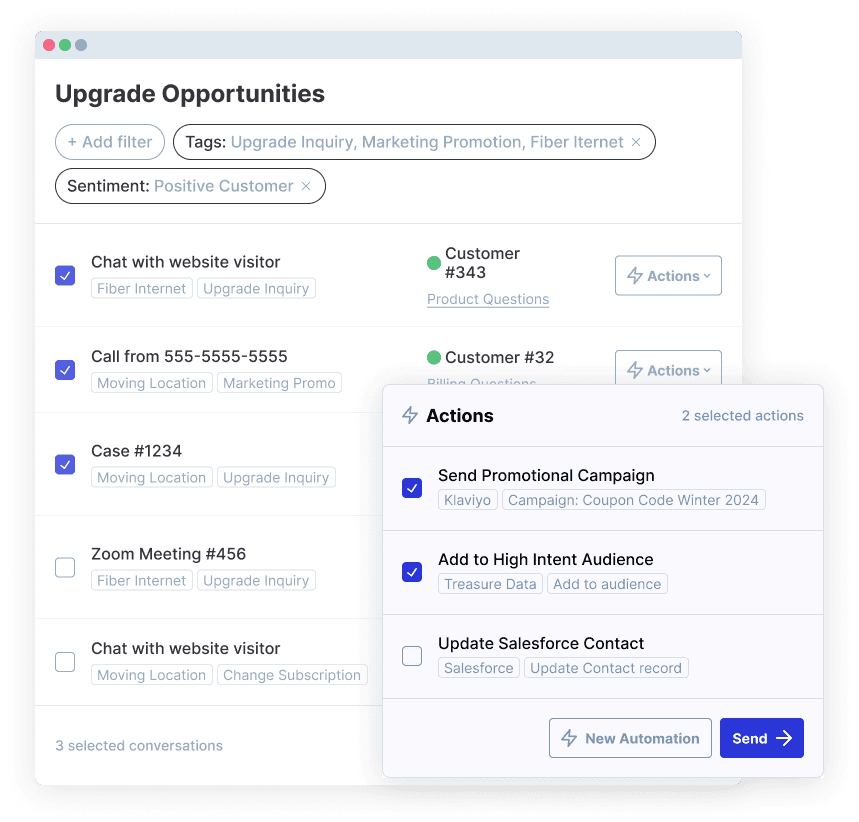
With automatic actions, insights can be integrated into a Customer Data Platform (CDP) to update individual customer profiles. For instance, if a customer frequently asks about a particular product feature, their profile can be tagged to indicate a specific interest in that feature. Using these updated profiles, dynamic audience segments can be created, such as "interested in new products," "requires customer support," or "high purchase intent."
Conversation Intelligence, and GenCI in particular, can be used to impact every layer of the business, not just the customer service org. From product to marketing to operations, business leaders can take action on key trends to prevent churn and drive growth. Here’s how.
Uncovering hidden customer insights
With analysis across every channel, businesses can get deep, detailed answers to unlock a new level of customer understanding. With generative insights, getting to the bottom of emerging issues is faster and enables customer service teams to more easily know what to do. If a customer expresses discontent around product quality, getting detailed information on the complaint, the specific product, and whether it’s a trend across several feedback channels can be surfaced in seconds.
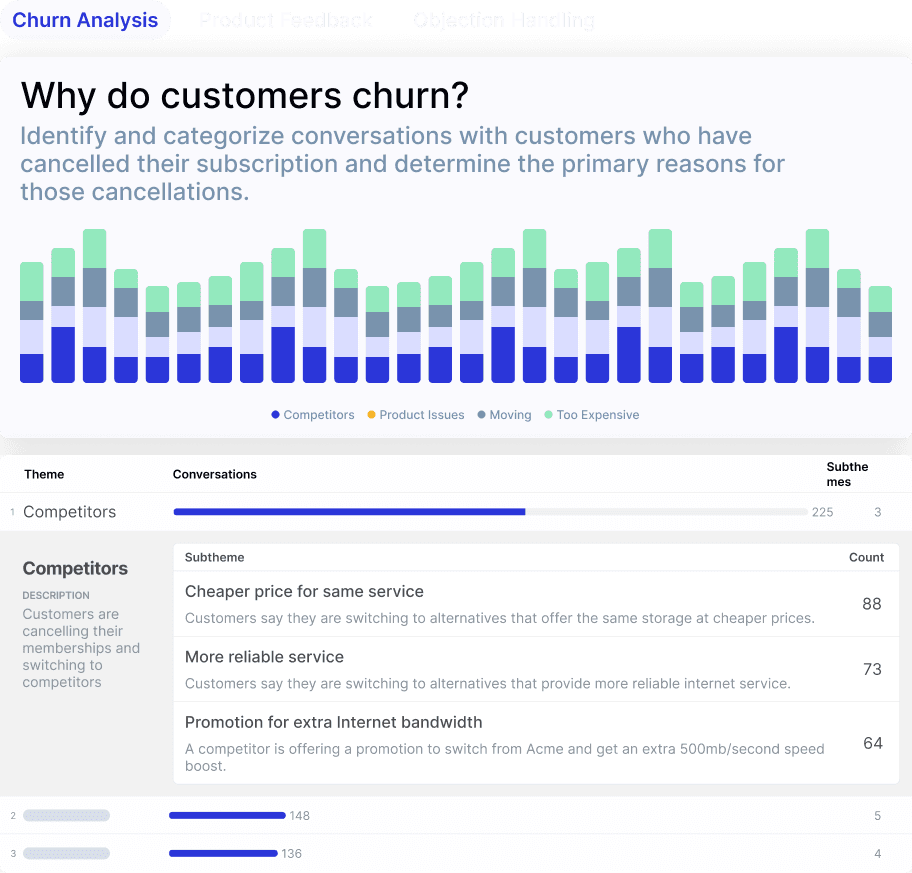
For example, Wine Enthusiast faced a surge in customer inquiries as a result of high demand for their at-home wine delivery services, which was straining their lean customer service team. They needed a better way to analyze customer conversations and act on them in the moment.
Using Echo AI, Wine Enthusiast discovered a trend in customer mentions that led them to discover a manufacturing defect that was causing rusting on electrical components. This insight helped Wine Enthusiast swiftly resolve the manufacturing issue to prevent any future customer churn, ensuring their quality standards were upheld.
Automating Manual Call Reviews
Harnessing AI and automation isn't just about cutting costs—it's about maximizing output.
By automating the most repetitive tasks in a customer service organization, employees can focus on the work that makes the biggest difference, rather than reviewing hundreds of calls manually, for instance.
With Echo AI's AutoQA capabilities, analyzing every customer interaction is completed within minutes. This eliminates the need for manual review, saving businesses valuable time previously spent identifying reasons behind call surges or complaints.
Conversational AI also helps to assess and score agents based on predefined criteria, such as:
Adherence to scripts
Professionalism
Resolution efficiency
Compliance with company policies
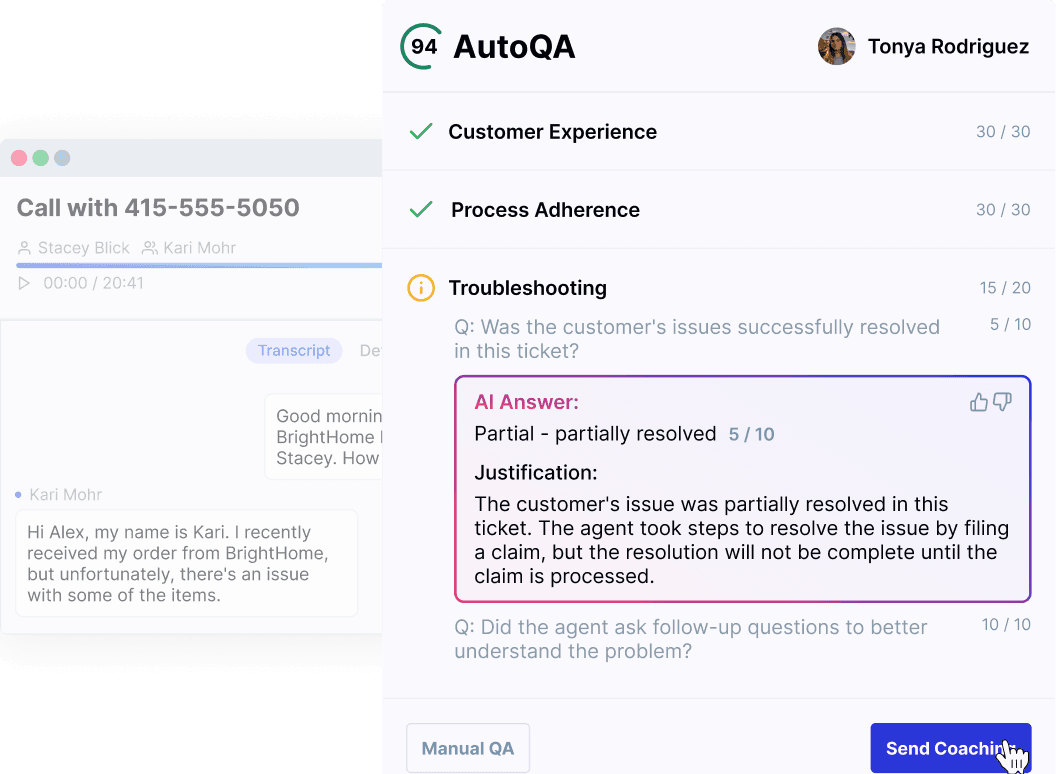
Generative insights are also used to automate quality scoring and act on insights, which also relieves the burden of having to communicate alerts manually across departments.
As an example, NativePath, a health and nutrition brand, was spending 75% of the week manually reviewing calls to glean insights into agent performance. It was a time-consuming process that hindered efficiency and productivity. Now, NativePath has made its review process quicker than ever, reducing time spent by 90% across the board.
Using Insights to Boost Campaigns
The value of genCI goes well beyond the customer service organization. Marketing teams, for example, can gain immense value from using customer data insights. As a function that is deeply tied to understanding the Voice of the Customer, marketing stands to gain immense value from chatbot interactions, calls, support tickets, and more to improve acquisition and lifecycle marketing efforts. (You can get a deeper dive on this here).
Generative insights can be used to improve how audiences are segmented, providing a way to optimize these cohorts at a much more data-driven level. GenCI can identify intent, or the key phrases, recurring topics, and sentiment indicators that reveal customer interests, concerns, and intentions.
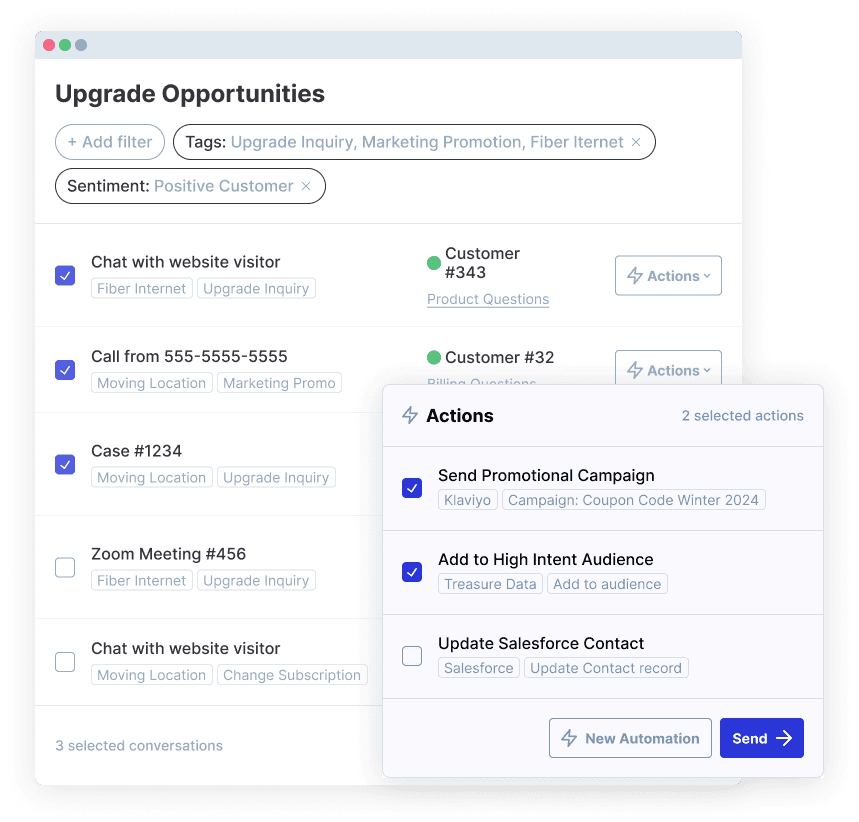
With automatic actions, insights can be integrated into a Customer Data Platform (CDP) to update individual customer profiles. For instance, if a customer frequently asks about a particular product feature, their profile can be tagged to indicate a specific interest in that feature. Using these updated profiles, dynamic audience segments can be created, such as "interested in new products," "requires customer support," or "high purchase intent."
Conversation Intelligence, and GenCI in particular, can be used to impact every layer of the business, not just the customer service org. From product to marketing to operations, business leaders can take action on key trends to prevent churn and drive growth. Here’s how.
Uncovering hidden customer insights
With analysis across every channel, businesses can get deep, detailed answers to unlock a new level of customer understanding. With generative insights, getting to the bottom of emerging issues is faster and enables customer service teams to more easily know what to do. If a customer expresses discontent around product quality, getting detailed information on the complaint, the specific product, and whether it’s a trend across several feedback channels can be surfaced in seconds.
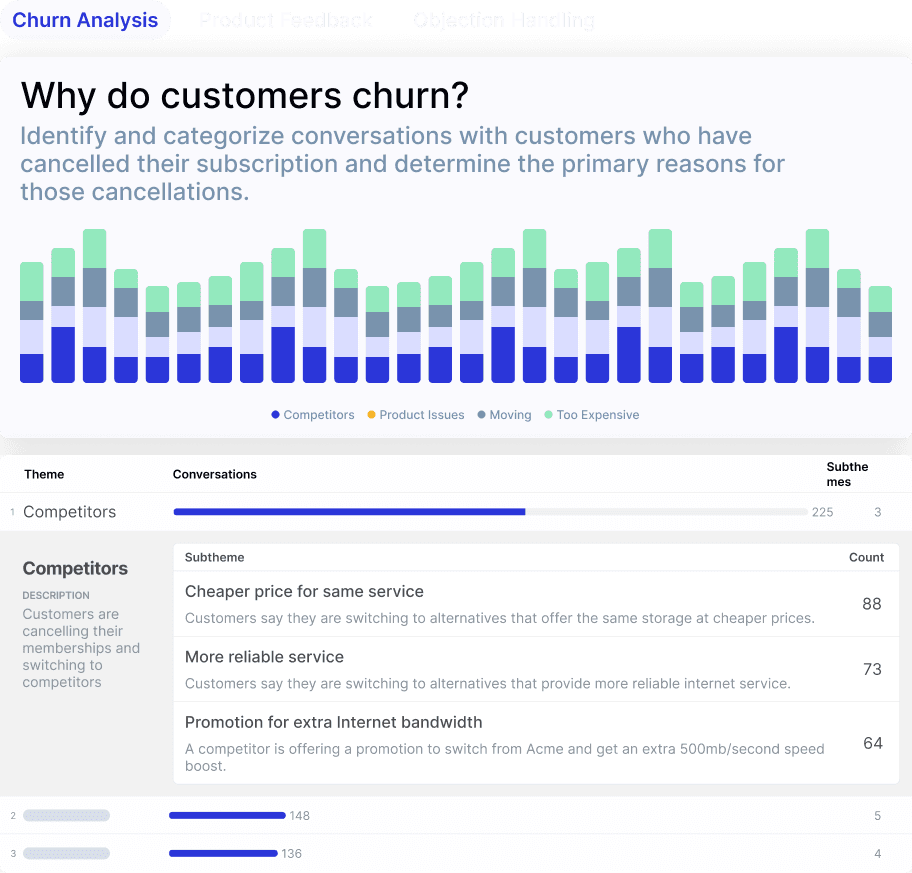
For example, Wine Enthusiast faced a surge in customer inquiries as a result of high demand for their at-home wine delivery services, which was straining their lean customer service team. They needed a better way to analyze customer conversations and act on them in the moment.
Using Echo AI, Wine Enthusiast discovered a trend in customer mentions that led them to discover a manufacturing defect that was causing rusting on electrical components. This insight helped Wine Enthusiast swiftly resolve the manufacturing issue to prevent any future customer churn, ensuring their quality standards were upheld.
Automating Manual Call Reviews
Harnessing AI and automation isn't just about cutting costs—it's about maximizing output.
By automating the most repetitive tasks in a customer service organization, employees can focus on the work that makes the biggest difference, rather than reviewing hundreds of calls manually, for instance.
With Echo AI's AutoQA capabilities, analyzing every customer interaction is completed within minutes. This eliminates the need for manual review, saving businesses valuable time previously spent identifying reasons behind call surges or complaints.
Conversational AI also helps to assess and score agents based on predefined criteria, such as:
Adherence to scripts
Professionalism
Resolution efficiency
Compliance with company policies
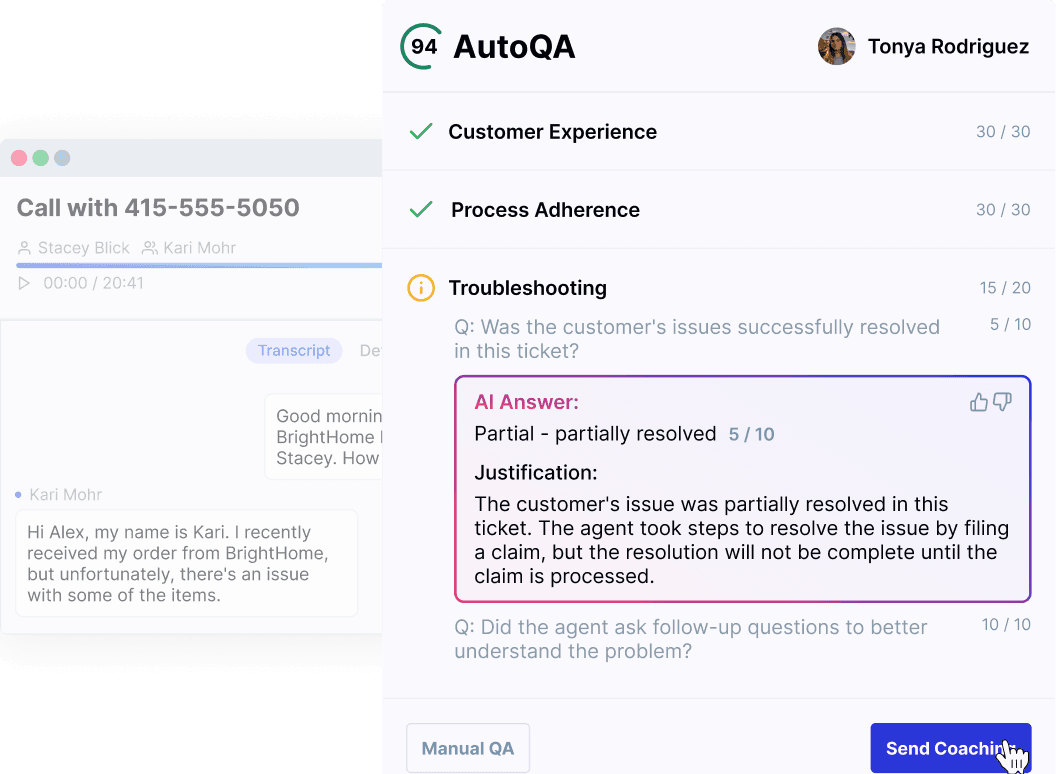
Generative insights are also used to automate quality scoring and act on insights, which also relieves the burden of having to communicate alerts manually across departments.
As an example, NativePath, a health and nutrition brand, was spending 75% of the week manually reviewing calls to glean insights into agent performance. It was a time-consuming process that hindered efficiency and productivity. Now, NativePath has made its review process quicker than ever, reducing time spent by 90% across the board.
Using Insights to Boost Campaigns
The value of genCI goes well beyond the customer service organization. Marketing teams, for example, can gain immense value from using customer data insights. As a function that is deeply tied to understanding the Voice of the Customer, marketing stands to gain immense value from chatbot interactions, calls, support tickets, and more to improve acquisition and lifecycle marketing efforts. (You can get a deeper dive on this here).
Generative insights can be used to improve how audiences are segmented, providing a way to optimize these cohorts at a much more data-driven level. GenCI can identify intent, or the key phrases, recurring topics, and sentiment indicators that reveal customer interests, concerns, and intentions.
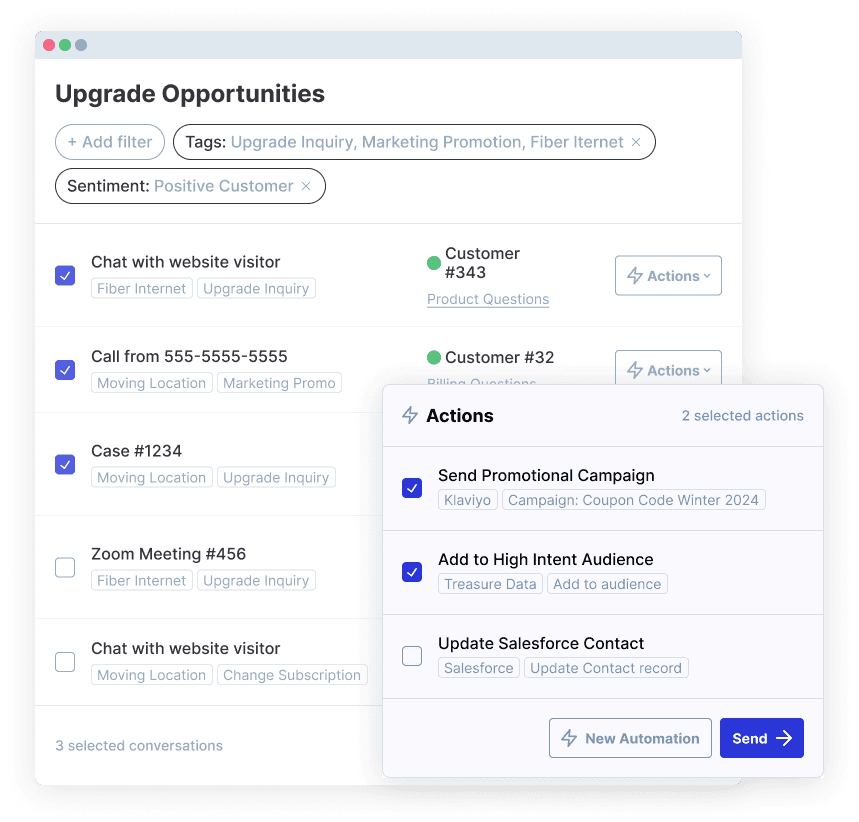
With automatic actions, insights can be integrated into a Customer Data Platform (CDP) to update individual customer profiles. For instance, if a customer frequently asks about a particular product feature, their profile can be tagged to indicate a specific interest in that feature. Using these updated profiles, dynamic audience segments can be created, such as "interested in new products," "requires customer support," or "high purchase intent."
Who Can Use Conversation Intelligence Software?
Conversation intelligence tools can be used by a wide range of professionals across a number of industries and business functions, namely go-to-market teams. Let's break this down.
Customer Service Teams
Traditionally, customer service teams, particularly those in the contact center, have used what used to be termed speech analytics solutions to monitor interactions, enhance response times, and reduce churn. These customer service teams, from VPs to managers and below, use conversation intelligence to uncover key moments in conversations, understand top reasons behind cancellations, key churn drivers, and top customer complaints during high season.
QA Teams
Many tools on the market today have fused conversation intelligence with things like QA monitoring and coaching to help grade agents and improve performance. QA analysts can use these types of software to automatically grade agents across 100% of calls to remove subjectivity from agent performance scoring. It also eliminates the need of having manual scorecards to track and identify top and bottom performing agents.
Sales Teams
Sales teams benefit from conversation intelligence by analyzing calls to identify successful strategies and improve pitches, having recordings of every sales conversation and then analyzed by top themes, categorizing common objections, and prospect sentiment/tonality in conversations. It's often used by sales enablement to identify training topics and focus on coaching sales reps in parts of the buying process where they struggle most. Most conversation intelligence software for sales focuses on things like reducing the time in the buying cycle, providing sales assistance, and using predictive analysis to determine which deals have the most likelihood to close.
Marketing Teams
As we learned about earlier in this blog, marketing teams can leverage generative insights to understand customer preferences and create more targeted campaigns, identifying high propensity leads and improving how they move across the funnel. Conversation intelligence can also be used to analyze social feeds and third party reviews to surface top themes and trends to use for campaigns, whether it's to re-engage lapsed customers or acquire new ones.
Conversation intelligence tools can be used by a wide range of professionals across a number of industries and business functions, namely go-to-market teams. Let's break this down.
Customer Service Teams
Traditionally, customer service teams, particularly those in the contact center, have used what used to be termed speech analytics solutions to monitor interactions, enhance response times, and reduce churn. These customer service teams, from VPs to managers and below, use conversation intelligence to uncover key moments in conversations, understand top reasons behind cancellations, key churn drivers, and top customer complaints during high season.
QA Teams
Many tools on the market today have fused conversation intelligence with things like QA monitoring and coaching to help grade agents and improve performance. QA analysts can use these types of software to automatically grade agents across 100% of calls to remove subjectivity from agent performance scoring. It also eliminates the need of having manual scorecards to track and identify top and bottom performing agents.
Sales Teams
Sales teams benefit from conversation intelligence by analyzing calls to identify successful strategies and improve pitches, having recordings of every sales conversation and then analyzed by top themes, categorizing common objections, and prospect sentiment/tonality in conversations. It's often used by sales enablement to identify training topics and focus on coaching sales reps in parts of the buying process where they struggle most. Most conversation intelligence software for sales focuses on things like reducing the time in the buying cycle, providing sales assistance, and using predictive analysis to determine which deals have the most likelihood to close.
Marketing Teams
As we learned about earlier in this blog, marketing teams can leverage generative insights to understand customer preferences and create more targeted campaigns, identifying high propensity leads and improving how they move across the funnel. Conversation intelligence can also be used to analyze social feeds and third party reviews to surface top themes and trends to use for campaigns, whether it's to re-engage lapsed customers or acquire new ones.
Conversation intelligence tools can be used by a wide range of professionals across a number of industries and business functions, namely go-to-market teams. Let's break this down.
Customer Service Teams
Traditionally, customer service teams, particularly those in the contact center, have used what used to be termed speech analytics solutions to monitor interactions, enhance response times, and reduce churn. These customer service teams, from VPs to managers and below, use conversation intelligence to uncover key moments in conversations, understand top reasons behind cancellations, key churn drivers, and top customer complaints during high season.
QA Teams
Many tools on the market today have fused conversation intelligence with things like QA monitoring and coaching to help grade agents and improve performance. QA analysts can use these types of software to automatically grade agents across 100% of calls to remove subjectivity from agent performance scoring. It also eliminates the need of having manual scorecards to track and identify top and bottom performing agents.
Sales Teams
Sales teams benefit from conversation intelligence by analyzing calls to identify successful strategies and improve pitches, having recordings of every sales conversation and then analyzed by top themes, categorizing common objections, and prospect sentiment/tonality in conversations. It's often used by sales enablement to identify training topics and focus on coaching sales reps in parts of the buying process where they struggle most. Most conversation intelligence software for sales focuses on things like reducing the time in the buying cycle, providing sales assistance, and using predictive analysis to determine which deals have the most likelihood to close.
Marketing Teams
As we learned about earlier in this blog, marketing teams can leverage generative insights to understand customer preferences and create more targeted campaigns, identifying high propensity leads and improving how they move across the funnel. Conversation intelligence can also be used to analyze social feeds and third party reviews to surface top themes and trends to use for campaigns, whether it's to re-engage lapsed customers or acquire new ones.
Conversation intelligence tools can be used by a wide range of professionals across a number of industries and business functions, namely go-to-market teams. Let's break this down.
Customer Service Teams
Traditionally, customer service teams, particularly those in the contact center, have used what used to be termed speech analytics solutions to monitor interactions, enhance response times, and reduce churn. These customer service teams, from VPs to managers and below, use conversation intelligence to uncover key moments in conversations, understand top reasons behind cancellations, key churn drivers, and top customer complaints during high season.
QA Teams
Many tools on the market today have fused conversation intelligence with things like QA monitoring and coaching to help grade agents and improve performance. QA analysts can use these types of software to automatically grade agents across 100% of calls to remove subjectivity from agent performance scoring. It also eliminates the need of having manual scorecards to track and identify top and bottom performing agents.
Sales Teams
Sales teams benefit from conversation intelligence by analyzing calls to identify successful strategies and improve pitches, having recordings of every sales conversation and then analyzed by top themes, categorizing common objections, and prospect sentiment/tonality in conversations. It's often used by sales enablement to identify training topics and focus on coaching sales reps in parts of the buying process where they struggle most. Most conversation intelligence software for sales focuses on things like reducing the time in the buying cycle, providing sales assistance, and using predictive analysis to determine which deals have the most likelihood to close.
Marketing Teams
As we learned about earlier in this blog, marketing teams can leverage generative insights to understand customer preferences and create more targeted campaigns, identifying high propensity leads and improving how they move across the funnel. Conversation intelligence can also be used to analyze social feeds and third party reviews to surface top themes and trends to use for campaigns, whether it's to re-engage lapsed customers or acquire new ones.
How Managers Can Use Conversation Intelligence
Managers, particularly in contact centers, can significantly enhance their day-to-day operations with this technology. Conversation intelligence tools allow them to analyze customer interactions in real-time, providing deep insights into common issues and areas where agents or sales reps may need additional training. By monitoring these interactions, managers can identify patterns in customer complaints, track performance metrics, and ensure compliance with company standards.
This leads to more targeted coaching and development programs, improving overall service quality. Additionally, conversation intelligence helps in predicting potential churn by highlighting frequent pain points and dissatisfaction, enabling proactive measures to retain customers. Overall, this technology empowers managers to make data-driven decisions that enhance both agent performance and customer satisfaction, helping them better report on what’s happening on the ground to C-level executives.
“Echo AI quickly morphed into really a team comparison and a departmental directional tool... More than just an individual coaching tool.” —Hugo Munday, Director of CX, ThriftBooks
Managers, particularly in contact centers, can significantly enhance their day-to-day operations with this technology. Conversation intelligence tools allow them to analyze customer interactions in real-time, providing deep insights into common issues and areas where agents or sales reps may need additional training. By monitoring these interactions, managers can identify patterns in customer complaints, track performance metrics, and ensure compliance with company standards.
This leads to more targeted coaching and development programs, improving overall service quality. Additionally, conversation intelligence helps in predicting potential churn by highlighting frequent pain points and dissatisfaction, enabling proactive measures to retain customers. Overall, this technology empowers managers to make data-driven decisions that enhance both agent performance and customer satisfaction, helping them better report on what’s happening on the ground to C-level executives.
“Echo AI quickly morphed into really a team comparison and a departmental directional tool... More than just an individual coaching tool.” —Hugo Munday, Director of CX, ThriftBooks
Managers, particularly in contact centers, can significantly enhance their day-to-day operations with this technology. Conversation intelligence tools allow them to analyze customer interactions in real-time, providing deep insights into common issues and areas where agents or sales reps may need additional training. By monitoring these interactions, managers can identify patterns in customer complaints, track performance metrics, and ensure compliance with company standards.
This leads to more targeted coaching and development programs, improving overall service quality. Additionally, conversation intelligence helps in predicting potential churn by highlighting frequent pain points and dissatisfaction, enabling proactive measures to retain customers. Overall, this technology empowers managers to make data-driven decisions that enhance both agent performance and customer satisfaction, helping them better report on what’s happening on the ground to C-level executives.
“Echo AI quickly morphed into really a team comparison and a departmental directional tool... More than just an individual coaching tool.” —Hugo Munday, Director of CX, ThriftBooks
Managers, particularly in contact centers, can significantly enhance their day-to-day operations with this technology. Conversation intelligence tools allow them to analyze customer interactions in real-time, providing deep insights into common issues and areas where agents or sales reps may need additional training. By monitoring these interactions, managers can identify patterns in customer complaints, track performance metrics, and ensure compliance with company standards.
This leads to more targeted coaching and development programs, improving overall service quality. Additionally, conversation intelligence helps in predicting potential churn by highlighting frequent pain points and dissatisfaction, enabling proactive measures to retain customers. Overall, this technology empowers managers to make data-driven decisions that enhance both agent performance and customer satisfaction, helping them better report on what’s happening on the ground to C-level executives.
“Echo AI quickly morphed into really a team comparison and a departmental directional tool... More than just an individual coaching tool.” —Hugo Munday, Director of CX, ThriftBooks
How Frontline Agents Can Use Conversation Intelligence
Frontline agents in customer service can greatly benefit from using conversation intelligence to better understand their performance and improve how they deliver customer service.
GenCI allows them to access real-time transcriptions and analysis of their interactions, providing immediate feedback on their performance and helping them adjust their communication strategies on the fly. Agents can quickly identify and respond to customer emotions and concerns by recognizing sentiment patterns, which enhances their ability to provide empathetic and effective service.
Some conversation intelligence tools also enable agents with faster access to knowledge management resources by surfacing relevant information and solutions real-time during a call, reducing the time spent searching for answers and improving first-call resolution rates. Additionally, tools can offer personalized coaching tips and highlight best practices based on previous interactions, enabling agents to continuously improve their skills and deliver superior customer experiences. Overall, conversation intelligence empowers frontline agents to be more responsive, informed, and effective in their roles, leading to higher customer satisfaction and operational efficiency.
Frontline agents in customer service can greatly benefit from using conversation intelligence to better understand their performance and improve how they deliver customer service.
GenCI allows them to access real-time transcriptions and analysis of their interactions, providing immediate feedback on their performance and helping them adjust their communication strategies on the fly. Agents can quickly identify and respond to customer emotions and concerns by recognizing sentiment patterns, which enhances their ability to provide empathetic and effective service.
Some conversation intelligence tools also enable agents with faster access to knowledge management resources by surfacing relevant information and solutions real-time during a call, reducing the time spent searching for answers and improving first-call resolution rates. Additionally, tools can offer personalized coaching tips and highlight best practices based on previous interactions, enabling agents to continuously improve their skills and deliver superior customer experiences. Overall, conversation intelligence empowers frontline agents to be more responsive, informed, and effective in their roles, leading to higher customer satisfaction and operational efficiency.
Frontline agents in customer service can greatly benefit from using conversation intelligence to better understand their performance and improve how they deliver customer service.
GenCI allows them to access real-time transcriptions and analysis of their interactions, providing immediate feedback on their performance and helping them adjust their communication strategies on the fly. Agents can quickly identify and respond to customer emotions and concerns by recognizing sentiment patterns, which enhances their ability to provide empathetic and effective service.
Some conversation intelligence tools also enable agents with faster access to knowledge management resources by surfacing relevant information and solutions real-time during a call, reducing the time spent searching for answers and improving first-call resolution rates. Additionally, tools can offer personalized coaching tips and highlight best practices based on previous interactions, enabling agents to continuously improve their skills and deliver superior customer experiences. Overall, conversation intelligence empowers frontline agents to be more responsive, informed, and effective in their roles, leading to higher customer satisfaction and operational efficiency.
Frontline agents in customer service can greatly benefit from using conversation intelligence to better understand their performance and improve how they deliver customer service.
GenCI allows them to access real-time transcriptions and analysis of their interactions, providing immediate feedback on their performance and helping them adjust their communication strategies on the fly. Agents can quickly identify and respond to customer emotions and concerns by recognizing sentiment patterns, which enhances their ability to provide empathetic and effective service.
Some conversation intelligence tools also enable agents with faster access to knowledge management resources by surfacing relevant information and solutions real-time during a call, reducing the time spent searching for answers and improving first-call resolution rates. Additionally, tools can offer personalized coaching tips and highlight best practices based on previous interactions, enabling agents to continuously improve their skills and deliver superior customer experiences. Overall, conversation intelligence empowers frontline agents to be more responsive, informed, and effective in their roles, leading to higher customer satisfaction and operational efficiency.
How to Evaluate Conversation Intelligence Vendors
Whether you’ve tried traditional contact center analytics tools in the past and have been let down by results, or you are ready to dip your feet into the water with AI for the first time, it's important to set clear evaluation criteria for what you need.
Our Buying Guide walks you through everything you need to know, including setting business requirements, checklists, and how to make the buying decision. Below are the key key evaluation criteria points to consider as you get started.
Technology - Choose a vendor that uses a multi-LLM approach
The power of a generative conversation intelligence platform lies in how accurate its insights are. That’s why it’s so important to partner with vendors who have built a system that taps into the power of dozens of LLMs, each designed for unique analysis points like specific business questions, intent, and more.
Data Inputs - Choose a vendor that ingests more data sources
When it comes to understanding your customer, the range of customer data being analyzed matters. More data sources mean a richer understanding of your customer, which can lead to better decision-making. This is where analyzing reviews, surveys, and even social media in addition to tickets and calls can elevate the quality of insights you are obtaining.
Workflows - Choose a vendor that ties insights to actions
Insights are only valuable if they’re put to use. Even with highly accurate learnings about your business, if you can’t apply them to improve every area of your company, you’re not making the best use of them. That’s why choosing a vendor that makes insights actionable, and impacts the broader organization, plays a role in getting the most out of your investment.
Proof of Concept - Choose a vendor that shows value with your own data
Testing with your own data allows you to assess the accuracy and effectiveness of the vendor you’re evaluating in real-world scenarios. A Proof of Concept provides valuable insights into how well the solution performs with your data and whether it meets your expectations. Seeing insights in action with your own data also builds confidence among your own internal stakeholders, including executives, users, and investors. It demonstrates capabilities and potential value, fostering buy-in and support for the final decision, maximizing its potential impact on your organization.
Customization - Choose a vendor that offers customized models for your unique needs
AI models adjusted with internal data tend to offer more accurate and relevant results. Custom models offer greater flexibility compared to off-the-shelf solutions, and they can be adapted and refined as your business needs evolve or as new challenges arise. Custom models are trained with your company's data, allowing them to recognize patterns and trends unique to the organization.
Integrations - Opt for a vendor with a strong integrations network
Extensive integrations mean customer and conversation data are synced seamlessly.A vendor with a robust integrations network can seamlessly integrate customer insights with your existing systems and workflows, ensuring insights are interconnected and sent to your data warehouse. By leveraging the capabilities of multiple tools through integrations, you can create more comprehensive solutions that address complex business requirements.
Implementation - Prioritize a vendor that implements in weeks, not months
Time to value is critical if you want your investment to impact your business in less time. Lengthy implementation schedules demand IT staffing efforts from your team, taking time away from meeting critical KPIs. Vendors that are built on newer generation technologies don’t require long time frames to go live–and if they do, the burden should not rest on your team’s shoulders.
White Glove Customer Success - Go with a people-first vendor
Choose trustworthy partners you can trust. When vendors prioritize people, they tend to focus on building long-term relationships based on trust, collaboration, and mutual respect. This can lead to more stable and beneficial partnerships over time, with both parties invested in each other's success.
Whether you’ve tried traditional contact center analytics tools in the past and have been let down by results, or you are ready to dip your feet into the water with AI for the first time, it's important to set clear evaluation criteria for what you need.
Our Buying Guide walks you through everything you need to know, including setting business requirements, checklists, and how to make the buying decision. Below are the key key evaluation criteria points to consider as you get started.
Technology - Choose a vendor that uses a multi-LLM approach
The power of a generative conversation intelligence platform lies in how accurate its insights are. That’s why it’s so important to partner with vendors who have built a system that taps into the power of dozens of LLMs, each designed for unique analysis points like specific business questions, intent, and more.
Data Inputs - Choose a vendor that ingests more data sources
When it comes to understanding your customer, the range of customer data being analyzed matters. More data sources mean a richer understanding of your customer, which can lead to better decision-making. This is where analyzing reviews, surveys, and even social media in addition to tickets and calls can elevate the quality of insights you are obtaining.
Workflows - Choose a vendor that ties insights to actions
Insights are only valuable if they’re put to use. Even with highly accurate learnings about your business, if you can’t apply them to improve every area of your company, you’re not making the best use of them. That’s why choosing a vendor that makes insights actionable, and impacts the broader organization, plays a role in getting the most out of your investment.
Proof of Concept - Choose a vendor that shows value with your own data
Testing with your own data allows you to assess the accuracy and effectiveness of the vendor you’re evaluating in real-world scenarios. A Proof of Concept provides valuable insights into how well the solution performs with your data and whether it meets your expectations. Seeing insights in action with your own data also builds confidence among your own internal stakeholders, including executives, users, and investors. It demonstrates capabilities and potential value, fostering buy-in and support for the final decision, maximizing its potential impact on your organization.
Customization - Choose a vendor that offers customized models for your unique needs
AI models adjusted with internal data tend to offer more accurate and relevant results. Custom models offer greater flexibility compared to off-the-shelf solutions, and they can be adapted and refined as your business needs evolve or as new challenges arise. Custom models are trained with your company's data, allowing them to recognize patterns and trends unique to the organization.
Integrations - Opt for a vendor with a strong integrations network
Extensive integrations mean customer and conversation data are synced seamlessly.A vendor with a robust integrations network can seamlessly integrate customer insights with your existing systems and workflows, ensuring insights are interconnected and sent to your data warehouse. By leveraging the capabilities of multiple tools through integrations, you can create more comprehensive solutions that address complex business requirements.
Implementation - Prioritize a vendor that implements in weeks, not months
Time to value is critical if you want your investment to impact your business in less time. Lengthy implementation schedules demand IT staffing efforts from your team, taking time away from meeting critical KPIs. Vendors that are built on newer generation technologies don’t require long time frames to go live–and if they do, the burden should not rest on your team’s shoulders.
White Glove Customer Success - Go with a people-first vendor
Choose trustworthy partners you can trust. When vendors prioritize people, they tend to focus on building long-term relationships based on trust, collaboration, and mutual respect. This can lead to more stable and beneficial partnerships over time, with both parties invested in each other's success.
Whether you’ve tried traditional contact center analytics tools in the past and have been let down by results, or you are ready to dip your feet into the water with AI for the first time, it's important to set clear evaluation criteria for what you need.
Our Buying Guide walks you through everything you need to know, including setting business requirements, checklists, and how to make the buying decision. Below are the key key evaluation criteria points to consider as you get started.
Technology - Choose a vendor that uses a multi-LLM approach
The power of a generative conversation intelligence platform lies in how accurate its insights are. That’s why it’s so important to partner with vendors who have built a system that taps into the power of dozens of LLMs, each designed for unique analysis points like specific business questions, intent, and more.
Data Inputs - Choose a vendor that ingests more data sources
When it comes to understanding your customer, the range of customer data being analyzed matters. More data sources mean a richer understanding of your customer, which can lead to better decision-making. This is where analyzing reviews, surveys, and even social media in addition to tickets and calls can elevate the quality of insights you are obtaining.
Workflows - Choose a vendor that ties insights to actions
Insights are only valuable if they’re put to use. Even with highly accurate learnings about your business, if you can’t apply them to improve every area of your company, you’re not making the best use of them. That’s why choosing a vendor that makes insights actionable, and impacts the broader organization, plays a role in getting the most out of your investment.
Proof of Concept - Choose a vendor that shows value with your own data
Testing with your own data allows you to assess the accuracy and effectiveness of the vendor you’re evaluating in real-world scenarios. A Proof of Concept provides valuable insights into how well the solution performs with your data and whether it meets your expectations. Seeing insights in action with your own data also builds confidence among your own internal stakeholders, including executives, users, and investors. It demonstrates capabilities and potential value, fostering buy-in and support for the final decision, maximizing its potential impact on your organization.
Customization - Choose a vendor that offers customized models for your unique needs
AI models adjusted with internal data tend to offer more accurate and relevant results. Custom models offer greater flexibility compared to off-the-shelf solutions, and they can be adapted and refined as your business needs evolve or as new challenges arise. Custom models are trained with your company's data, allowing them to recognize patterns and trends unique to the organization.
Integrations - Opt for a vendor with a strong integrations network
Extensive integrations mean customer and conversation data are synced seamlessly.A vendor with a robust integrations network can seamlessly integrate customer insights with your existing systems and workflows, ensuring insights are interconnected and sent to your data warehouse. By leveraging the capabilities of multiple tools through integrations, you can create more comprehensive solutions that address complex business requirements.
Implementation - Prioritize a vendor that implements in weeks, not months
Time to value is critical if you want your investment to impact your business in less time. Lengthy implementation schedules demand IT staffing efforts from your team, taking time away from meeting critical KPIs. Vendors that are built on newer generation technologies don’t require long time frames to go live–and if they do, the burden should not rest on your team’s shoulders.
White Glove Customer Success - Go with a people-first vendor
Choose trustworthy partners you can trust. When vendors prioritize people, they tend to focus on building long-term relationships based on trust, collaboration, and mutual respect. This can lead to more stable and beneficial partnerships over time, with both parties invested in each other's success.
Whether you’ve tried traditional contact center analytics tools in the past and have been let down by results, or you are ready to dip your feet into the water with AI for the first time, it's important to set clear evaluation criteria for what you need.
Our Buying Guide walks you through everything you need to know, including setting business requirements, checklists, and how to make the buying decision. Below are the key key evaluation criteria points to consider as you get started.
Technology - Choose a vendor that uses a multi-LLM approach
The power of a generative conversation intelligence platform lies in how accurate its insights are. That’s why it’s so important to partner with vendors who have built a system that taps into the power of dozens of LLMs, each designed for unique analysis points like specific business questions, intent, and more.
Data Inputs - Choose a vendor that ingests more data sources
When it comes to understanding your customer, the range of customer data being analyzed matters. More data sources mean a richer understanding of your customer, which can lead to better decision-making. This is where analyzing reviews, surveys, and even social media in addition to tickets and calls can elevate the quality of insights you are obtaining.
Workflows - Choose a vendor that ties insights to actions
Insights are only valuable if they’re put to use. Even with highly accurate learnings about your business, if you can’t apply them to improve every area of your company, you’re not making the best use of them. That’s why choosing a vendor that makes insights actionable, and impacts the broader organization, plays a role in getting the most out of your investment.
Proof of Concept - Choose a vendor that shows value with your own data
Testing with your own data allows you to assess the accuracy and effectiveness of the vendor you’re evaluating in real-world scenarios. A Proof of Concept provides valuable insights into how well the solution performs with your data and whether it meets your expectations. Seeing insights in action with your own data also builds confidence among your own internal stakeholders, including executives, users, and investors. It demonstrates capabilities and potential value, fostering buy-in and support for the final decision, maximizing its potential impact on your organization.
Customization - Choose a vendor that offers customized models for your unique needs
AI models adjusted with internal data tend to offer more accurate and relevant results. Custom models offer greater flexibility compared to off-the-shelf solutions, and they can be adapted and refined as your business needs evolve or as new challenges arise. Custom models are trained with your company's data, allowing them to recognize patterns and trends unique to the organization.
Integrations - Opt for a vendor with a strong integrations network
Extensive integrations mean customer and conversation data are synced seamlessly.A vendor with a robust integrations network can seamlessly integrate customer insights with your existing systems and workflows, ensuring insights are interconnected and sent to your data warehouse. By leveraging the capabilities of multiple tools through integrations, you can create more comprehensive solutions that address complex business requirements.
Implementation - Prioritize a vendor that implements in weeks, not months
Time to value is critical if you want your investment to impact your business in less time. Lengthy implementation schedules demand IT staffing efforts from your team, taking time away from meeting critical KPIs. Vendors that are built on newer generation technologies don’t require long time frames to go live–and if they do, the burden should not rest on your team’s shoulders.
White Glove Customer Success - Go with a people-first vendor
Choose trustworthy partners you can trust. When vendors prioritize people, they tend to focus on building long-term relationships based on trust, collaboration, and mutual respect. This can lead to more stable and beneficial partnerships over time, with both parties invested in each other's success.
Get the Buying Guide
Conversation Intelligence offers deep, powerful insight into which levers to pull to deliver top-notch experiences across every layer of the organization.
With countless solutions on the market today, it can be hard to decide which approach makes the most sense for your business needs, and where to even get started. We’ve got you covered.
Conversation Intelligence offers deep, powerful insight into which levers to pull to deliver top-notch experiences across every layer of the organization.
With countless solutions on the market today, it can be hard to decide which approach makes the most sense for your business needs, and where to even get started. We’ve got you covered.
Conversation Intelligence offers deep, powerful insight into which levers to pull to deliver top-notch experiences across every layer of the organization.
With countless solutions on the market today, it can be hard to decide which approach makes the most sense for your business needs, and where to even get started. We’ve got you covered.
Conversation Intelligence offers deep, powerful insight into which levers to pull to deliver top-notch experiences across every layer of the organization.
With countless solutions on the market today, it can be hard to decide which approach makes the most sense for your business needs, and where to even get started. We’ve got you covered.
From the blog
From the blog
From the blog
From the blog
Solutions
© 2024 All Right Reserved by Echo AI
Solutions
© 2024 All Right Reserved by Echo AI
Solutions
© 2024 All Right Reserved by Echo AI
Solutions
© 2024 All Right Reserved by Echo AI